Medical image interpretation is undergoing a transformative shift thanks to advancements in artificial intelligence (AI), and LEARNS.EDU.VN is dedicated to bringing you the latest insights in this rapidly evolving field. A generalist learner, such as the innovative MedVersa model, promises to revolutionize how we approach multifaceted medical image interpretation by offering versatile solutions that can adapt to various tasks. Dive into the future of medical diagnostics, where AI empowers healthcare professionals with comprehensive tools for enhanced patient care and learn how integrated AI systems are set to redefine diagnostic accuracy and broaden clinical applicability, utilizing synergistic algorithms for complex medical data analysis.
1. Understanding the Landscape of Medical AI
The world of medical Artificial Intelligence has been rapidly evolving, promising a new age of accuracy in diagnostics as well as overall patient care. Researchers have primarily been focused on developing AI solutions for the performance of specific tasks. However, the AI systems currently in place often find themselves restricted to more narrow applications. This limits their broader adoption in everyday clinical practice. These systems typically struggle with adaptability and integration which are essential for comprehensive diagnostic solutions.
- Specialized AI Models: excel in specific tasks like detecting certain types of anomalies in X-rays but lack the adaptability to handle diverse imaging modalities.
- Limitations: present significant challenges in real-world clinical settings where a variety of imaging data needs interpretation.
- Potential Solutions: Innovative models such as MedVersa that are created for multifaceted interpretation hold the potential to bridge this gap through adaptable as well as integrated AI.
2. Introducing MedVersa: A Generalist AI Model
To combat current limitations, a team of researchers from Harvard Medical School, Jawaharlal Institute of Postgraduate Medical Education and Research, along with Scripps Research Translational Institute, have come together to propose MedVersa. MedVersa is an AI model created to enable adaptive learning along with tasking for medical image interpretation.
- Key Features of MedVersa
- Designed for multifaceted learning and versatile application.
- Aims to tackle current limitations in medical AI.
- Offers an adaptable learning and tasking approach for medical image interpretation.
- The Core Innovation
- MedVersa utilizes a large language model as a learnable orchestrator.
- The orchestrator integrates multimodal inputs.
- Executes tasks using both language and vision modules.
- Overcoming Traditional Limitations
- Combines visual and linguistic supervision during learning.
- Supports on-the-fly task specification through language.
- Enables training on diverse medical data across modalities and tasks.
- Results in general, shared representations that improve the AI’s adaptability and accuracy.
3. The Architecture and Functionality of MedVersa
MedVersa is designed to revolutionize medical image interpretation through its unique architecture, which allows for more adaptable learning and precise tasking. The basic model lies in its use of a large language model to act as a learnable orchestrator, which combines multimodal inputs and completes tasks with language as well as vision modules.
3.1. The Role of the Large Language Model
The large language model (LLM) works as the brain of MedVersa, coordinating the diverse data inputs along with outputs. The LLM integrates both language and vision modules, allowing MedVersa to carry out tasks with a thorough understanding of medical contexts.
This architecture enables MedVersa to train on various medical data, spanning various modalities along with tasks, producing more generalized and shared representations.
3.2. Multimodal Input Integration
MedVersa has the ability to integrate different types of medical data, including imaging data, clinical notes, and patient history. This multimodal approach is crucial because it enables the AI to learn from a wide range of data. By looking at various data, MedVersa can make more accurate and comprehensive interpretations, which is critical for complex medical diagnostics.
3.3. Task Execution Through Language and Vision Modules
MedVersa’s power lies in its ability to use language to specify tasks as they happen. This means users are able to ask specific questions or ask for specific analysis, and MedVersa adapts in real time. For example, a radiologist could ask MedVersa to “identify any fractures in this X-ray” or “summarize the key findings from this MRI.” The combination of language and vision modules allows MedVersa to not only understand the task but also to execute it accurately.
4. MedVersa’s Versatility in Medical Image Interpretation
MedVersa is a versatile AI model which excels in vision-language tasks like generating radiology reports along with answering visual questions, and vision-centric challenges which include detecting anatomical structures along with segmenting medical images. This dual capability allows MedVersa to train using diverse medical data across multiple modalities as well as tasks, and that results in general shared representations.
4.1. Vision-Language Tasks
MedVersa shines in tasks that require both vision and language understanding. These tasks are vital for a thorough understanding of medical images, enabling healthcare professionals to gain more insights and improve decision-making.
- Radiology Report Generation
- MedVersa can automatically generate detailed radiology reports from medical images.
- Reports include descriptions of findings, interpretations, and recommendations.
- Speeds up the reporting process and reduces the workload on radiologists.
- Visual Question Answering
- MedVersa can answer specific questions about medical images.
- Provides immediate insights and helps in quick decision-making.
- Enhances the efficiency of diagnostic processes.
4.2. Vision-Centric Challenges
In addition to vision-language tasks, MedVersa also excels in challenges that rely primarily on visual data. These capabilities are crucial for precise medical imaging analysis and the detection of subtle abnormalities.
- Anatomical Structure Detection
- MedVersa can accurately identify and locate anatomical structures in medical images.
- Assists in the identification of key landmarks and potential abnormalities.
- Enhances the precision of diagnostic evaluations.
- Medical Image Segmentation
- MedVersa can segment medical images to isolate specific regions or structures of interest.
- Enables detailed analysis and measurement of anatomical features.
- Supports quantitative assessments and monitoring of disease progression.
5. The MedInterp Dataset: Fueling MedVersa’s Development
The creation of MedVersa was supported by the curation of a diverse, multimodal dataset referred to as MedInterp, which was specifically created for multifaceted medical image interpretation. The training and assessment of MedVersa with the MedInterp dataset showed that it goes above and beyond the state-of-the-art specialist counterparts in nine tasks.
5.1. Significance of a Diverse Dataset
A diverse dataset is crucial for training a generalist AI model such as MedVersa. It ensures that the model is exposed to a wide range of medical imaging scenarios, improving its ability to generalize and perform well across different tasks and modalities.
- Comprehensive Coverage:
- Includes images from various anatomical regions.
- Features a wide range of pathologies and abnormalities.
- Incorporates data from different imaging modalities (X-ray, MRI, CT scans, etc.).
- Enhanced Generalization:
- Enables MedVersa to learn robust and transferable features.
- Reduces the risk of overfitting to specific datasets or tasks.
- Improves performance on unseen data and real-world clinical scenarios.
5.2. Key Components of the MedInterp Dataset
The MedInterp dataset comprises a variety of medical images and associated annotations, carefully curated to facilitate comprehensive training and evaluation of MedVersa.
Component | Description |
---|---|
Medical Images | Includes a wide variety of medical images from different modalities (X-ray, MRI, CT scans, ultrasound, etc.) and anatomical regions. The images cover various pathologies and abnormalities, ensuring a comprehensive representation of medical imaging scenarios. |
Annotations | Provides detailed annotations for each image, including bounding boxes, segmentations, labels, and textual descriptions. These annotations are essential for training MedVersa to accurately detect anatomical structures, segment regions of interest, and generate informative reports. |
Clinical Notes | Contains clinical notes and patient histories associated with the medical images. These notes provide additional context and information, enabling MedVersa to learn more nuanced relationships between imaging findings and clinical outcomes. |
Task Specifications | Includes task specifications for various medical image interpretation tasks, such as radiology report generation, visual question answering, and anatomical structure detection. These specifications guide the training process and ensure that MedVersa can perform a wide range of tasks with high accuracy. |
Validation and Test Sets | Consists of separate validation and test sets to evaluate MedVersa’s performance and generalization capabilities. These sets are carefully curated to ensure that they are representative of real-world clinical scenarios and can provide reliable measures of MedVersa’s effectiveness. |
Longitudinal Study Data | Incorporates data from longitudinal studies, allowing MedVersa to learn how to track changes and trends over time. This is particularly useful for monitoring disease progression and evaluating the effectiveness of treatments. |
Region-of-Interest Captioning | Includes regions of interest (ROIs) along with captions describing the relevant findings. This component enables MedVersa to generate descriptive summaries of specific areas within medical images, enhancing its ability to communicate key insights to healthcare professionals. |
Open-Ended Visual QA | Features open-ended questions about the images, challenging MedVersa to understand and reason about the visual content in a comprehensive manner. This component promotes more advanced learning and helps MedVersa to provide nuanced and informative answers. |
Chest Pathology Classification | Provides data for classifying chest pathologies, such as pneumonia, lung cancer, and tuberculosis. This component enables MedVersa to assist in the early detection and diagnosis of common respiratory conditions, improving patient outcomes. |
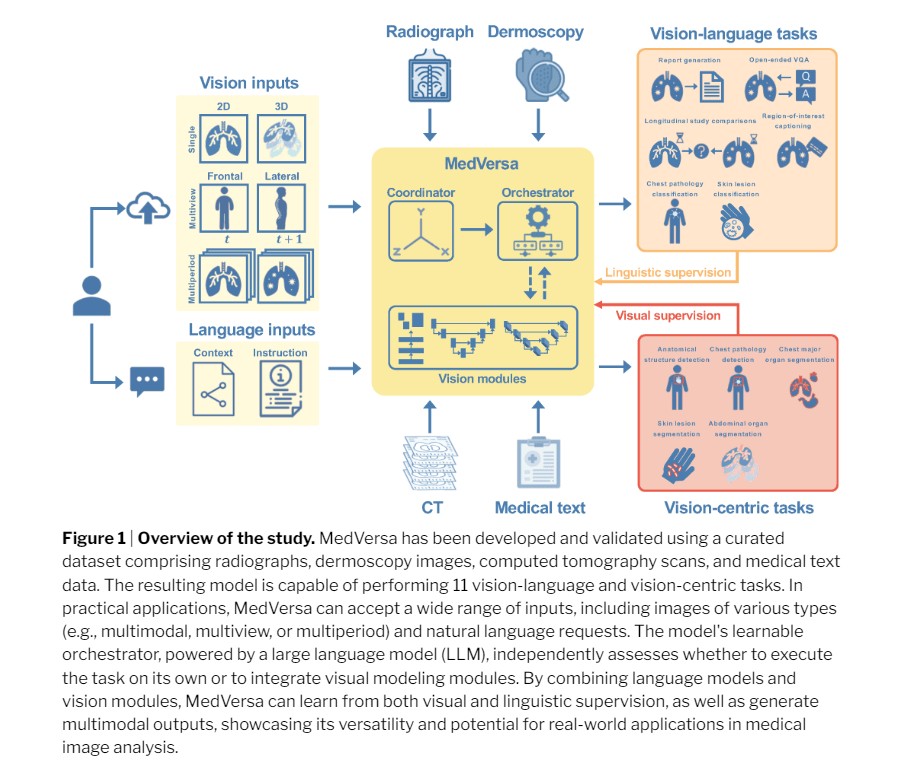
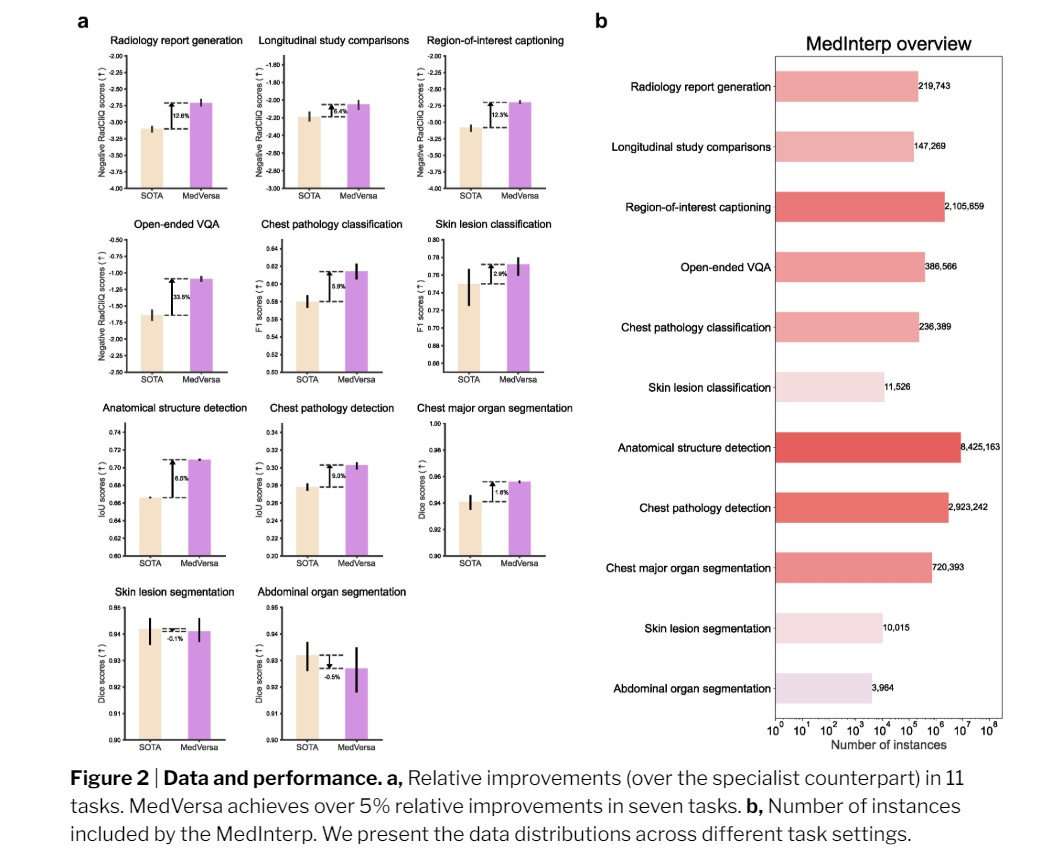
6. Performance Benchmarks and Comparative Analysis
MedVersa’s performance has been rigorously tested against state-of-the-art specialist models, demonstrating its superior capabilities in various medical image interpretation tasks. The model’s ability to outperform these specialized counterparts underscores its potential as a versatile tool in clinical practice.
6.1. Outperforming Specialist Models
MedVersa has demonstrated exceptional performance across a wide array of tasks, often surpassing the capabilities of specialist models that are designed for specific functions. This is due to MedVersa’s ability to generalize from diverse data along with apply the learned information effectively across different tasks.
6.2. Specific Task Achievements
In radiology report generation, MedVersa surpassed MAIRA-1 21, which is a specialist multimodal model from Microsoft, and Med-PaLM M 13, a generalist biomedical foundation model from Google that is ten times larger than MedVersa. MedVersa also excelled in visual localization tasks, surpassing a well-established object detector in localization tasks. MedVersa showed superior performance compared to state-of-the-art specialist methods in other tasks including longitudinal study comparisons, region-of-interest captioning, open-ended visual question answering, along with chest pathology classification.
7. Real-World Applications and Clinical Implications
The development of MedVersa opens up various possibilities for real-world applications, ultimately transforming medical image interpretation and patient care. Its versatility and superior performance in multifaceted tasks make it a valuable tool for healthcare professionals across different specialties.
7.1. Enhancing Diagnostic Accuracy
MedVersa improves diagnostic accuracy with the capacity to look at multimodal inputs and perform thorough image analysis. By integrating visual and linguistic data, MedVersa is able to catch subtle patterns and irregularities that might be missed by human observers or more specialized AI models.
7.2. Streamlining Clinical Workflows
The use of MedVersa is able to streamline clinical workflows, saving time and resources for healthcare providers. Its ability to automatically generate radiology reports, answer visual questions, and detect anatomical structures all aid in lowering the manual workload, enabling radiologists as well as other medical professionals to focus on more complicated tasks along with patient care.
7.3. Supporting Personalized Medicine
MedVersa supports personalized medicine by giving more tailored and detailed insights into patient conditions. By looking at longitudinal study data and tracking changes over time, MedVersa can assist in more informed treatment decisions that are based on the unique characteristics and progression of a patient’s disease.
8. MedVersa as a Pioneer in Generalist Medical AI (GMAI)
Researchers believe that MedVersa is the first generalist medical AI model to support multimodal inputs, outputs, along with on-the-fly task specification. The creation of MedVersa has the potential to unlock new chances for building more versatile GMAI models.
8.1. Defining GMAI
Generalist Medical AI (GMAI) represents a paradigm shift from specialized AI models that are designed for specific tasks. GMAI models such as MedVersa are engineered to perform a wide array of tasks with high accuracy, making them versatile tools for healthcare professionals.
8.2. Advantages of GMAI
GMAI models offer several advantages over traditional specialized AI models. These benefits stem from their ability to generalize across different tasks, modalities, and datasets, leading to more efficient and effective clinical applications.
- Versatility:
- Can perform a wide range of tasks, including image analysis, report generation, and clinical decision support.
- Adapts to different imaging modalities and anatomical regions.
- Reduces the need for multiple specialized AI models.
- Efficiency:
- Streamlines clinical workflows by automating multiple tasks.
- Saves time and resources for healthcare providers.
- Improves the speed and accuracy of diagnostic processes.
- Scalability:
- Can be easily scaled to handle large volumes of data.
- Supports the integration of new tasks and modalities.
- Adapts to evolving clinical needs and technological advancements.
8.3. Future Directions for GMAI
The creation of MedVersa serves as a springboard for future GMAI model development, and there are numerous chances for improvement and expansion. By addressing current constraints and investigating new methods, GMAI models can potentially change healthcare and patient care.
- Improved Generalization:
- Develop models that can generalize across even more diverse datasets and tasks.
- Incorporate data from different patient populations and clinical settings.
- Enhance the robustness and reliability of GMAI models in real-world scenarios.
- Enhanced Interpretability:
- Create models that provide transparent and interpretable explanations for their decisions.
- Enable healthcare professionals to understand the reasoning behind AI predictions.
- Promote trust and acceptance of GMAI models in clinical practice.
- Seamless Integration:
- Integrate GMAI models with existing healthcare IT systems.
- Enable seamless data exchange and workflow automation.
- Support the development of comprehensive clinical decision support systems.
9. Ethical Considerations and Responsible AI Development
As AI becomes more and more integrated into healthcare, it is critical to address ethical considerations along with guaranteeing responsible AI development. These considerations include privacy, bias, transparency, and accountability, all of which are important for maintaining the integrity and trustworthiness of AI systems.
9.1. Data Privacy and Security
Protecting patient data is of the utmost importance in medical AI applications. AI developers need to adhere to strict data privacy regulations and use strong security measures to protect sensitive information from unauthorized access and breaches.
9.2. Addressing Bias in AI Models
AI models are able to inherit biases from the data that they are trained on, which could result in unfair or discriminatory outcomes. To solve this issue, it is crucial to carefully curate datasets to guarantee that they are diverse and representative of different patient populations.
9.3. Ensuring Transparency and Explainability
Transparency and explainability are vital for building trust in AI systems. Healthcare professionals need to understand how AI models make decisions, and they need to be able to evaluate the reasoning behind AI predictions.
9.4. Accountability and Oversight
Accountability and oversight systems must be established to guarantee that AI systems are being used ethically and responsibly. This includes establishing clear lines of responsibility for AI-related decisions, and establishing processes for monitoring as well as auditing AI performance.
10. How LEARNS.EDU.VN Supports Your Learning Journey
At LEARNS.EDU.VN, we are dedicated to providing comprehensive educational resources and insights into the latest advancements in medical AI. Whether you are a healthcare professional, a student, or simply interested in the field, our platform offers a wealth of knowledge and opportunities to enhance your understanding.
10.1. Access to Expert Articles and Resources
LEARNS.EDU.VN offers a wide range of expert articles and resources on medical AI, covering everything from the fundamentals to the latest breakthroughs. Our content is carefully curated to provide accurate, up-to-date, and accessible information for learners of all levels.
10.2. Comprehensive Courses and Training Programs
We provide comprehensive courses and training programs that are created to improve your knowledge as well as skills in medical AI. Whether you are wanting to learn about medical image analysis, machine learning, or data science, our courses are created to satisfy your needs.
- Medical Image Analysis: Gain expertise in the techniques and tools used to analyze medical images, including segmentation, registration, and classification.
- Machine Learning in Healthcare: Learn how to apply machine learning algorithms to solve real-world healthcare problems, such as disease diagnosis, treatment planning, and patient monitoring.
- Data Science for Medical Professionals: Develop your data science skills and learn how to extract valuable insights from medical data, including electronic health records, clinical trial data, and genomic data.
10.3. Connecting with a Community of Learners
LEARNS.EDU.VN also gives you the chance to connect with a community of learners, experts, along with professionals in the field of medical AI. Our forums and discussion boards provide a platform for sharing knowledge, asking questions, along with collaborating on projects.
FAQ: Generalist Learner for Multifaceted Medical Image Interpretation
-
What is a generalist learner in the context of medical image interpretation?
A generalist learner is an AI model designed to perform a wide range of tasks related to medical image interpretation, unlike specialized models that focus on specific tasks.
-
How does MedVersa differ from traditional AI models in medical imaging?
MedVersa uses a large language model as a learnable orchestrator, integrating multimodal inputs and executing tasks with both language and vision modules, enabling more adaptable learning and precise tasking.
-
What is the MedInterp dataset, and why is it important?
MedInterp is a diverse, multimodal dataset specifically designed for multifaceted medical image interpretation, which supports the training and assessment of MedVersa, ensuring it outperforms state-of-the-art specialist counterparts.
-
What are some real-world applications of MedVersa in healthcare?
MedVersa can enhance diagnostic accuracy, streamline clinical workflows, support personalized medicine, generate radiology reports, answer visual questions, detect anatomical structures, and assist in longitudinal study comparisons.
-
What are the ethical considerations in developing GMAI models like MedVersa?
Ethical considerations include ensuring data privacy and security, addressing bias in AI models, ensuring transparency and explainability, and establishing accountability and oversight systems.
-
How can healthcare professionals benefit from using MedVersa?
Healthcare professionals can benefit from improved diagnostic accuracy, streamlined workflows, more informed treatment decisions, and the ability to focus on more complex tasks and patient care.
-
What are the future directions for GMAI (Generalist Medical AI) development?
Future directions include improving generalization across diverse datasets, enhancing interpretability of AI decisions, and seamless integration with existing healthcare IT systems.
-
How does MedVersa handle different modalities of medical images (e.g., X-ray, MRI, CT scans)?
MedVersa is designed to integrate and analyze data from different imaging modalities, enabling it to learn from a wide range of data and make more accurate and comprehensive interpretations.
-
What makes MedVersa better than other generalist biomedical foundation models like Google’s Med-PaLM M?
MedVersa has shown superior performance in tasks such as radiology report generation and visual localization, despite being significantly smaller than models like Med-PaLM M.
-
Where can I find more information about MedVersa and its applications?
You can find more information about MedVersa on arXiv and explore related resources and courses on LEARNS.EDU.VN.
Conclusion
The development of generalist learners like MedVersa marks a crucial turning point in medical image interpretation, offering versatility, improved accuracy, and streamlined workflows. As AI continues to evolve, platforms such as LEARNS.EDU.VN provide healthcare professionals and learners with the resources needed to stay at the forefront of these advancements.
Are you ready to explore the possibilities of AI in medicine? Visit LEARNS.EDU.VN today to discover our expert articles, comprehensive courses, and a vibrant community of learners. Contact us at 123 Education Way, Learnville, CA 90210, United States, or via Whatsapp at +1 555-555-1212. Start your journey toward mastering multifaceted medical image interpretation with learns.edu.vn!