Generative Ai Vs Machine Learning, two transformative technologies, often get confused, but understanding their differences is crucial for navigating the AI landscape; Machine learning focuses on learning from data to make predictions or decisions, while generative AI goes a step further by creating new content. At LEARNS.EDU.VN, you can explore tailored courses that clarify these concepts and help you master these cutting-edge technologies, improving your skills and expanding your career options. Dive into the world of artificial intelligence and discover how both machine learning and generative models can revolutionize industries with our comprehensive programs, offering insights and practical skills in AI applications, model training, and data analysis.
1. Understanding Machine Learning Fundamentals
Machine learning (ML) is a subset of artificial intelligence that focuses on enabling systems to learn from data, identify patterns, and make decisions or predictions with minimal human intervention. This approach empowers machines to improve their performance over time as they are exposed to more data, enhancing their ability to make accurate assessments and automate complex tasks.
1.1. How Machine Learning Algorithms Work
At its core, machine learning involves feeding vast amounts of data into algorithms that can analyze this information and learn from it. The process typically includes:
- Data Collection: Gathering relevant data from various sources that the model will learn from.
- Training: Using the collected data to teach the model to recognize patterns, correlations, and insights.
- Validation and Testing: Ensuring the model performs well with new, unseen data to evaluate its accuracy and reliability.
Machine learning can be categorized into several types, including supervised, unsupervised, and semi-supervised learning.
- Supervised Learning: In supervised learning, models are trained on labeled data, where the input data is paired with the correct output, allowing the model to learn the mapping between inputs and outputs.
- Unsupervised Learning: Unsupervised learning deals with unlabeled data, where the model tries to identify patterns and relationships within the data on its own, without explicit guidance.
- Semi-Supervised Learning: This approach combines both labeled and unlabeled data, leveraging the benefits of both supervised and unsupervised techniques to improve model performance.
1.2. Key Applications of Machine Learning in Daily Life
Machine learning has become an integral part of our daily lives, with applications spanning various industries and domains. Here are some notable examples:
- Predictive Models in Finance: Algorithms analyze market trends, historical data, and economic indicators to forecast stock prices, assess credit risks, and detect fraudulent transactions.
- Recommendation Systems: Platforms like Netflix and Amazon use ML to suggest movies, products, or books based on your past behavior, preferences, and browsing history.
- Healthcare Diagnostics: Machine learning aids in diagnosing diseases by analyzing medical images, patient records, and genetic data more efficiently and accurately than human doctors.
2. Delving into Generative AI Innovations
Generative AI is a class of artificial intelligence that goes beyond analyzing data to create new content, be it text, images, music, or even video, that mimics human creations. Instead of merely making decisions or predictions based on input data, generative AI can generate novel data that wasn’t explicitly programmed into it.
2.1. Unpacking How Generative AI Functions
Generative AI models often utilize neural networks, particularly a type known as Generative Adversarial Networks (GANs), Variational Autoencoders (VAEs) or Large Language Models (LLMs). Here’s a simplified breakdown of the process:
- LLMs: These models, like GPT-4o, LLAMA, or Google Gemini, are trained on vast amounts of text data and can generate human-like text by predicting the next word in a sentence based on the context provided by previous words. They excel in tasks like language translation, content creation, and conversational agents.
- GANs: GANs consist of two neural networks, a generator and a discriminator, that work against each other. The generator creates new data instances while the discriminator evaluates them. Through this adversarial process, the generator improves its ability to produce realistic data.
- VAEs: VAEs use probabilistic models to generate new data, allowing for the creation of diverse and novel outputs based on learned representations of the input data.
2.2. Illustrative Examples of Generative AI in Action
Generative AI is increasingly popular and versatile, with applications spanning various creative and practical domains. Some notable examples include:
- Chatbots and Virtual Assistants: Tools like ChatGPT can generate human-like text based on the prompts they receive, making customer service interactions more natural and engaging.
- Deepfake Technology: AI can create highly realistic video and audio recordings that appear to be real, raising both exciting possibilities and ethical concerns.
- Art and Music: AI-generated art and music compositions offer new avenues for creativity, enabling artists and musicians to explore innovative ideas.
3. Essential Differences: Machine Learning vs Generative AI
While machine learning and generative AI are both subsets of artificial intelligence, their primary distinction lies in their purpose and output.
3.1. Core Purpose and Objectives
- Machine Learning: Focuses on understanding and predicting based on existing data, enabling machines to make accurate decisions or predictions.
- Generative AI: Geared towards creating new data that mimics human creations, pushing the boundaries of creativity and innovation.
3.2. Type of Output Produced
- Machine Learning: Outputs decisions or predictions, such as classifying data points, forecasting trends, or identifying anomalies.
- Generative AI: Produces new content, such as text, images, music, or videos, that didn’t exist before.
3.3. Key Applications and Use Cases
- Machine Learning: Used for tasks like recommendation systems, predictive analytics, fraud detection, and diagnostic tools.
- Generative AI: Employed in creative domains, deepfakes, content generation, drug discovery, and advanced simulations.
Feature | Machine Learning | Generative AI |
---|---|---|
Core Purpose | Understanding and predicting from existing data | Creating new data that mimics human creations |
Output Type | Decisions or predictions | New content (text, images, music, etc.) |
Key Applications | Recommendation systems, predictive analytics | Creative domains, deepfakes, content generation |
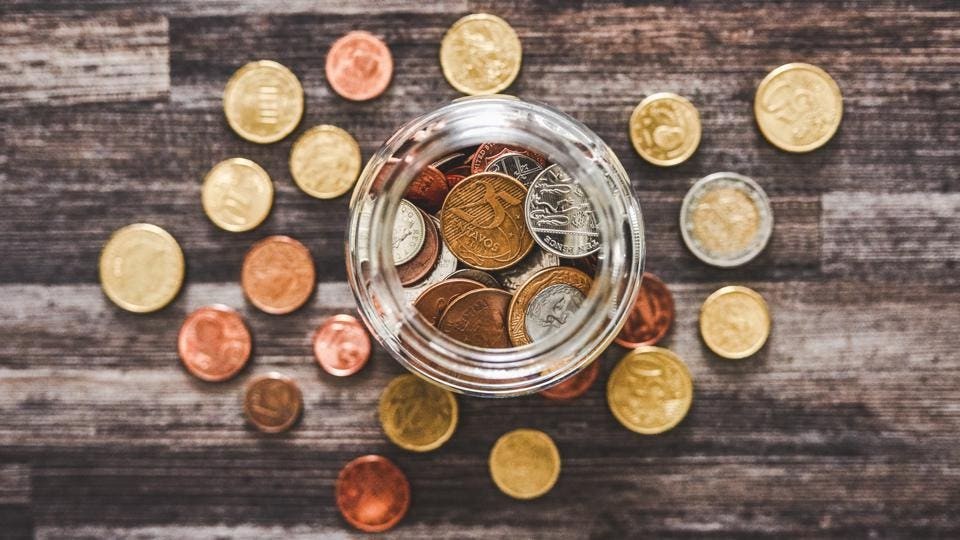
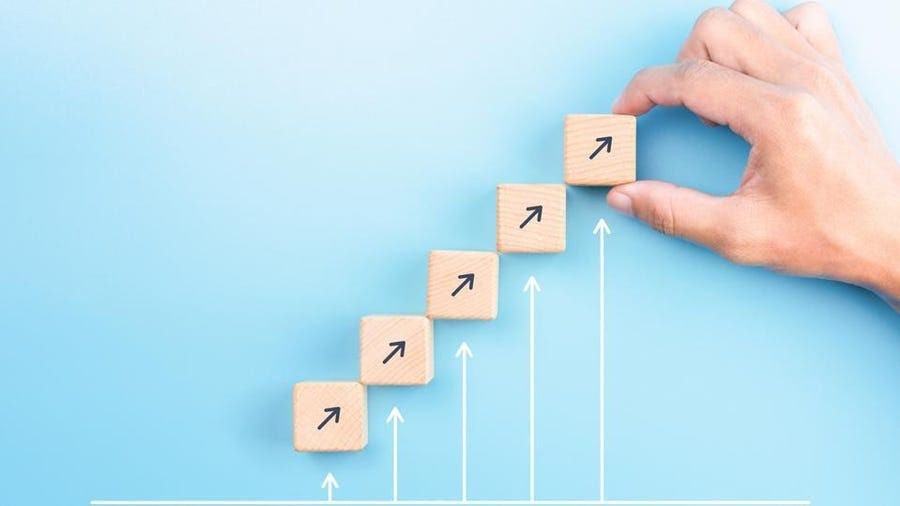
4. Exploring the Synergy Between ML and Generative AI
Despite their differences, machine learning and generative AI can complement each other in powerful ways, leading to enhanced capabilities and innovative applications.
4.1. Enhancing Generative Models with ML Techniques
Machine learning algorithms can improve the performance of generative AI models by providing better training data, refining the evaluation process, and optimizing model parameters. For example, machine learning can be used to preprocess and clean data, select relevant features, and identify biases in the training data, leading to more accurate and reliable generative models.
4.2. Utilizing Generative AI to Augment ML Training
Conversely, generative AI can enhance machine learning by creating synthetic data to train models in scenarios where real-world data is scarce or expensive to obtain. Generative models can generate realistic synthetic data that closely resembles real data, allowing machine learning models to learn more effectively and generalize better to unseen data.
4.3. Examples of Integrated ML and Generative AI Systems
Several applications demonstrate the synergy between machine learning and generative AI, including:
- Image and Video Generation: Generative models can create realistic images and videos from scratch, while machine learning can be used to enhance the quality, resolution, and diversity of the generated content.
- Natural Language Processing: Generative models can generate human-like text for chatbots, content creation, and language translation, while machine learning can be used to understand the intent, sentiment, and context of the generated text.
- Drug Discovery: Generative models can design novel drug candidates with desired properties, while machine learning can be used to predict their efficacy, safety, and interactions with other drugs.
5. The Future of AI: Collaboration and Innovation
As AI continues to evolve, the lines between machine learning and generative AI may blur, leading to even more sophisticated and versatile systems. Businesses and industries are already leveraging these technologies to drive innovation, enhance productivity, and create new customer experiences.
5.1. Emerging Trends in AI Development
- AI-Driven Automation: AI is increasingly being used to automate repetitive and mundane tasks, freeing up human workers to focus on more creative and strategic activities.
- Personalized Experiences: AI is enabling businesses to deliver personalized experiences to their customers, tailoring products, services, and content to their individual needs and preferences.
- Ethical AI: There is growing emphasis on developing AI systems that are fair, transparent, and accountable, ensuring that they do not perpetuate biases or discriminate against certain groups of people.
5.2. Industry Applications and Transformations
- Healthcare: Machine learning can predict patient outcomes and suggest treatments, while generative AI can create personalized medical content or simulate potential drug interactions.
- Entertainment: Machine learning curates content based on user preferences, while generative AI produces new music or art pieces tailored to individual tastes.
- Finance: Machine learning detects fraudulent transactions and assesses credit risks, while generative AI creates realistic simulations of financial markets.
5.3. Continuous Learning and Adaptation
To stay ahead in the rapidly evolving field of AI, continuous learning and adaptation are essential. Professionals need to acquire new skills, update their knowledge, and embrace new technologies to remain competitive and relevant in the job market. LEARNS.EDU.VN offers a wide range of courses, workshops, and resources to help individuals and organizations stay up-to-date with the latest trends and advancements in AI.
6. Case Studies: Real-World Impact of ML and Generative AI
Let’s explore a few case studies that highlight the transformative impact of machine learning and generative AI across different industries.
6.1. Healthcare: AI-Powered Diagnostics
A leading hospital implemented a machine learning system to analyze medical images, such as X-rays and MRIs, to detect diseases like cancer and pneumonia. The system was trained on a vast dataset of medical images and achieved an accuracy rate of over 95%, significantly improving the speed and accuracy of diagnoses.
6.2. Finance: Fraud Detection with Machine Learning
A major credit card company deployed a machine learning algorithm to detect fraudulent transactions in real-time. The algorithm analyzes transaction patterns, customer behavior, and other relevant data to identify suspicious activities and prevent fraud, saving the company millions of dollars each year.
6.3. Entertainment: AI-Generated Music
A music streaming platform used generative AI to create personalized music playlists tailored to individual user preferences. The AI system analyzes users’ listening history, mood, and activity level to generate unique playlists that match their tastes and preferences, enhancing their overall listening experience.
7. Ethical Considerations and Challenges
As AI technologies become more powerful and pervasive, it’s essential to address the ethical considerations and challenges associated with their use.
7.1. Bias and Fairness in AI Systems
AI systems can perpetuate biases present in the data they are trained on, leading to unfair or discriminatory outcomes. It’s crucial to ensure that AI systems are trained on diverse and representative datasets and that their algorithms are designed to mitigate biases.
7.2. Privacy and Data Security
AI systems often require access to vast amounts of personal data, raising concerns about privacy and data security. It’s essential to implement robust data protection measures, such as encryption, anonymization, and access controls, to safeguard sensitive information.
7.3. Transparency and Explainability
AI systems can be complex and opaque, making it difficult to understand how they arrive at their decisions or predictions. It’s essential to develop AI systems that are transparent and explainable, allowing users to understand the reasoning behind their outputs and hold them accountable for their actions.
8. Practical Steps to Get Started with AI
If you’re interested in getting started with AI, here are some practical steps you can take:
8.1. Learning Resources and Online Courses
- Online Platforms: Explore platforms like Coursera, edX, and Udacity for courses on machine learning, deep learning, and generative AI.
- Books and Tutorials: Read books and tutorials on AI concepts, algorithms, and programming languages like Python and R.
- Research Papers: Stay up-to-date with the latest research papers and publications in the field of AI.
8.2. Tools and Technologies
- Programming Languages: Learn Python and R, which are widely used in AI development.
- Machine Learning Frameworks: Familiarize yourself with frameworks like TensorFlow, Keras, and PyTorch.
- Cloud Platforms: Utilize cloud platforms like Amazon Web Services (AWS), Microsoft Azure, and Google Cloud Platform (GCP) for AI development and deployment.
8.3. Building Projects and Experimenting
- Start Small: Begin with small projects and gradually increase the complexity as you gain more experience.
- Experiment: Don’t be afraid to experiment with different algorithms, techniques, and datasets to see what works best.
- Collaborate: Join online communities and collaborate with other AI enthusiasts to learn from each other and share your knowledge.
9. The Role of LEARNS.EDU.VN in AI Education
LEARNS.EDU.VN plays a crucial role in AI education by providing comprehensive courses, resources, and support to individuals and organizations interested in learning about AI.
9.1. Comprehensive AI Courses and Programs
LEARNS.EDU.VN offers a wide range of AI courses and programs covering various topics, including machine learning, deep learning, natural language processing, and computer vision. These courses are designed to cater to learners of all levels, from beginners to advanced practitioners.
9.2. Expert Instructors and Mentors
LEARNS.EDU.VN partners with expert instructors and mentors who have years of experience in the field of AI. These instructors provide guidance, support, and mentorship to learners, helping them to achieve their learning goals.
9.3. Community and Networking Opportunities
LEARNS.EDU.VN fosters a vibrant community of AI learners, providing opportunities for networking, collaboration, and knowledge sharing. Learners can connect with each other, participate in discussions, and collaborate on projects, enhancing their learning experience.
10. Future Trends and Predictions in AI
The field of AI is constantly evolving, with new trends and technologies emerging all the time. Here are some future trends and predictions in AI:
10.1. AI in Edge Computing
AI is increasingly being deployed on edge devices, such as smartphones, drones, and IoT devices, enabling real-time data processing and decision-making without relying on cloud connectivity.
10.2. AI-Powered Cybersecurity
AI is being used to enhance cybersecurity by detecting and preventing cyber threats, analyzing network traffic, and automating security operations.
10.3. AI in Space Exploration
AI is playing a crucial role in space exploration by enabling autonomous navigation, data analysis, and resource management on space missions.
Understanding the difference between machine learning and generative AI is crucial for grasping the full scope of AI’s impact on our world. While machine learning excels at analyzing data and making predictions, generative AI pushes the boundaries of creativity by generating new and innovative content. Both technologies are reshaping industries, enhancing our daily lives, and opening up exciting possibilities for the future. As we continue to explore their potential, the collaboration between machine learning and generative AI will undoubtedly drive the next wave of technological advancements.
As AI continues to evolve, these advancements hold immense potential for transforming industries, enhancing productivity, and improving our daily lives. Stay curious, stay informed, and continue to explore the fascinating world of AI with LEARNS.EDU.VN.
FAQ: Generative AI vs Machine Learning
-
What is the main difference between generative AI and machine learning?
Generative AI creates new content, while machine learning focuses on making predictions or decisions based on existing data.
-
Can machine learning and generative AI be used together?
Yes, machine learning can enhance generative AI by improving training data, and generative AI can augment machine learning by creating synthetic training data.
-
What are some real-world applications of machine learning?
Recommendation systems, predictive analytics in finance, and healthcare diagnostics are common applications.
-
What are some examples of generative AI in action?
Chatbots, deepfake technology, and AI-generated art and music are notable examples.
-
How can I get started with AI?
Take online courses, learn programming languages like Python, and experiment with machine learning frameworks.
-
What is the role of LEARNS.EDU.VN in AI education?
LEARNS.EDU.VN provides comprehensive AI courses, expert instructors, and community networking opportunities.
-
What are some ethical considerations in AI development?
Addressing bias, ensuring data privacy, and promoting transparency are crucial ethical considerations.
-
What future trends can we expect in AI?
AI in edge computing, AI-powered cybersecurity, and AI in space exploration are emerging trends.
-
How can AI be used in healthcare?
AI can predict patient outcomes, suggest treatments, and create personalized medical content.
-
Where can I find reliable AI learning resources?
LEARNS.EDU.VN offers a wide range of courses, workshops, and resources to help you learn about AI.
Unlock your potential in the world of AI with LEARNS.EDU.VN! Explore our comprehensive courses and resources to master machine learning and generative AI. Visit our website learns.edu.vn to discover the perfect learning path for you. Contact us at 123 Education Way, Learnville, CA 90210, United States, or reach out via Whatsapp at +1 555-555-1212. Start your AI journey today!