Can Anyone Learn Data Science? Absolutely! Data science is a field accessible to anyone with the drive and willingness to learn. LEARNS.EDU.VN offers a structured path to mastering data science, regardless of your background, by focusing on practical skills and real-world projects. Unlock your potential and embark on a rewarding journey into data science, leveraging AI-driven insights and ethical data practices with our expert guidance and comprehensive resources.
1. Is Data Science Right For You?
The allure of data science lies in its potential to solve complex problems and extract meaningful insights from data. But is it a field that anyone can truly master? Let’s explore this question and understand the path to becoming a data scientist.
1.1. Is Data Science Still A Good Career Choice In Today’s World?
Yes, data science remains an excellent career choice. The demand for skilled data scientists continues to surge as organizations across various industries recognize the immense value of data-driven decision-making. According to a report by the U.S. Bureau of Labor Statistics, employment in computer and information research science occupations, including data science, is projected to grow 23 percent from 2022 to 2032, much faster than the average for all occupations. This growth is fueled by the increasing volume of data generated daily and the need for professionals who can effectively analyze and interpret it. This presents a large and growing opportunity for anyone who wants to learn data science.
1.2. Will AI Replace Data Scientists Or Change The Landscape Of The Field?
AI is unlikely to completely replace data scientists, but it will undoubtedly transform the role. The integration of AI into data science workflows will automate certain tasks and augment human capabilities, allowing data scientists to focus on more strategic and creative aspects of their work. Data scientists will increasingly collaborate with AI engineers and machine learning specialists to develop and fine-tune AI models, ensuring ethical considerations and addressing potential biases. This shift will require data scientists to acquire new skills in areas such as machine learning, deep learning, and natural language processing to remain competitive. With the support of LEARNS.EDU.VN, you’ll learn the most up-to-date skills.
1.3. What Key AI Skills Are Essential For Aspiring Data Scientists?
To thrive in the evolving landscape of data science, aspiring professionals must acquire a range of AI-related skills. These include a strong understanding of machine learning concepts, proficiency in deep learning frameworks like TensorFlow and PyTorch, expertise in natural language processing (NLP) for text analysis, and a deep understanding of AI ethics and bias mitigation. Data scientists should also be familiar with AI development tools and libraries, possess data engineering skills, and thrive in interdisciplinary, collaborative environments. Continuous learning and adaptation are crucial to stay abreast of the latest advancements in AI.
2. The Path To Data Science Mastery: A Step-By-Step Guide
The journey to becoming a data scientist can seem daunting, but by following a structured approach and focusing on practical skills, anyone can achieve their goals. Here’s a step-by-step guide to help you embark on your data science journey.
2.1. Step 1: Cultivate A Questioning Mindset
Data science is all about answering questions and uncovering insights using data and code. To excel in this field, you need to develop an analytical mindset and the ability to formulate meaningful questions. Start by analyzing news articles and data-driven reports, questioning the conclusions, and exploring the underlying data.
2.1.1. Analyzing News Articles And Data-Driven Reports
Begin by selecting news articles or reports that discuss data or statistical findings. Some examples include articles on health trends, economic indicators, or social issues. As you read, consider the following questions:
- How did the authors reach their conclusions based on the data presented?
- What assumptions did they make in their analysis?
- How could you design a study to investigate the topic further?
- What additional questions would you ask if you had access to the underlying data?
For instance, consider an article discussing the correlation between exercise and mental health. You might question the methodology used to collect the data, the sample size, and potential confounding factors. You could also explore whether different types of exercise have varying effects on mental well-being.
2.1.2. Exploring Available Datasets
Some articles and reports provide access to the underlying datasets used in their analysis. This allows you to delve deeper into the data and conduct your own investigations.
- Download the data and open it in a spreadsheet program like Excel or Google Sheets.
- Examine the data for patterns and trends.
- Do the data support the conclusions presented in the article or report? Why or why not?
- What additional questions can you answer using the data?
For example, if you find a dataset on gun deaths in the U.S., you could explore the data to identify trends in gun violence, analyze demographic factors associated with gun deaths, and examine the effectiveness of different gun control policies.
2.1.3. Good Places To Find Data-Driven Articles
- FiveThirtyEight: This website offers data-driven journalism on a variety of topics, including politics, sports, and science.
- The Upshot (New York Times): This section of the New York Times provides data-driven analysis of current events and social trends.
- Kaggle: This platform hosts data science competitions and datasets, often accompanied by articles and tutorials.
- Google Dataset Search: This search engine allows you to find datasets from various sources across the web.
- Data Is Plural: This weekly newsletter curates interesting datasets from around the web.
2.1.4. Reflecting On The Process
After several weeks of analyzing articles and exploring datasets, take time to reflect on the process. Did you enjoy formulating questions and seeking answers using data? Are you passionate about using data to solve problems and gain insights? If so, data science may be a good fit for you.
Data scientists constantly come up with questions and answer them using mathematical models and data analysis tools, so this step is great for understanding whether you’ll actually like the work.
2.1.5. Analyzing What You Enjoy
If you find the process of coming up with questions in the abstract challenging, try focusing on topics you’re passionate about. If you’re interested in health, analyze health data. If you’re interested in finance, analyze financial data. The key is to view your passions with an analytical mindset. If you want to put in the months of hard work necessary to learn data science, working on something you’re passionate about will help you stay motivated when you face setbacks.
2.2. Step 2: Master The Fundamentals
Once you’ve developed a questioning mindset, it’s time to acquire the technical skills needed to answer your questions. Start by learning the basics of programming in Python, a versatile language widely used in data science.
2.2.1. Choosing The Right Programming Language
Python is an excellent choice for beginners due to its clear syntax and extensive libraries for data analysis and machine learning. While other languages like R and Java are also used in data science, Python is often preferred for its versatility and ease of use.
2.2.2. Key Points To Remember
- Data science is about answering questions and driving business value, not about tools.
- Learning the concepts is more important than learning the syntax.
- Building projects and sharing them is what you’ll do in an actual data science role, and learning this way will give you a head start.
Super important note: The goal isn’t to learn everything; it’s to learn just enough to start building projects.
2.2.3. Essential Python Libraries For Data Science
- NumPy: A library for numerical computing, providing support for arrays and mathematical operations.
- Pandas: A library for data manipulation and analysis, offering data structures like DataFrames for organizing and analyzing data.
- Matplotlib: A library for creating visualizations, allowing you to generate charts, graphs, and plots to explore data.
- Scikit-learn: A library for machine learning, providing tools for classification, regression, clustering, and model evaluation.
2.2.4. Where To Learn Python
- Online Courses: Platforms like Codecademy, Coursera, and edX offer comprehensive Python courses for beginners.
- Interactive Tutorials: Websites like Dataquest and Learn Python provide interactive tutorials and coding exercises.
- Books: “Python Crash Course” by Eric Matthes and “Automate the Boring Stuff with Python” by Al Sweigart are excellent resources for learning Python.
- LEARNS.EDU.VN: Get access to our own comprehensive courses and a guided path to take you from beginner to expert!
2.2.5. Setting Up Your Development Environment
To write and run Python code, you’ll need to set up a development environment. This typically involves installing Python, a code editor, and any necessary libraries.
- Install Python: Download the latest version of Python from the official Python website (https://www.python.org/downloads/).
- Choose A Code Editor: Select a code editor that suits your preferences. Popular options include Visual Studio Code, Sublime Text, and Atom.
- Install Libraries: Use pip, the Python package installer, to install the essential data science libraries:
pip install numpy pandas matplotlib scikit-learn
2.2.6. Starting Your Coding Journey
Begin by writing simple Python programs to familiarize yourself with the language’s syntax and data structures. As you progress, tackle more complex coding challenges and explore the capabilities of the data science libraries. Remember to focus on building a solid foundation in programming concepts before diving into advanced topics.
2.3. Step 3: Build A Portfolio Of Data Science Projects
As you learn the basics of coding, start building projects to showcase your skills and demonstrate your ability to solve real-world problems using data science.
2.3.1. Finding Interesting Datasets
The key to building compelling data science projects is to find interesting datasets that align with your interests and passions.
- Public Data Repositories: Websites like Kaggle, UCI Machine Learning Repository, and Google Dataset Search offer a wide variety of datasets for various domains.
- Government Data Portals: Government agencies often publish data on topics like demographics, economics, and health.
- APIs: Many companies and organizations provide APIs that allow you to access their data programmatically.
2.3.2. Asking Questions About The Data
Once you’ve found a dataset, start asking questions about the data. What patterns and trends can you identify? What insights can you gain from the data? What questions can you answer using data analysis techniques?
2.3.3. Answering Questions With Code
Use your programming skills and data science libraries to answer the questions you’ve formulated. Clean and preprocess the data, perform exploratory data analysis, build machine learning models, and visualize your results.
2.3.4. Project Ideas For Beginners
- Analyzing Super Bowl Winners: Analyze data on Super Bowl winners to find patterns in team performance, player statistics, and game outcomes.
- Predicting Housing Prices: Build a machine learning model to predict housing prices based on features like location, size, and amenities.
- Analyzing Customer Reviews: Analyze customer reviews from online retailers to identify trends in customer sentiment and product satisfaction.
- Visualizing Traffic Patterns: Analyze traffic data to visualize traffic patterns and identify areas of congestion.
2.3.5. Key Considerations When Building Projects
- Most data science work is data cleaning.
- The most common machine learning technique is linear regression.
- Everyone starts somewhere. Even if you feel like what you’re doing isn’t impressive, it’s still worth working on.
2.3.6. Resources For Project Ideas
- Dataquest Projects: Dataquest’s courses contain interactive projects that you can complete while you’re learning.
- Kaggle Competitions: Participate in Kaggle competitions to tackle challenging data science problems and compete with other data scientists.
- Online Tutorials: Search for online tutorials and blog posts that provide step-by-step instructions for building data science projects.
2.3.7. Adding Project Complexity
After building a few small projects, it’s time to add layers of complexity to learn more advanced topics. As your skills grow, you can make the problem more complex by adding nuances like minute-by-minute prices and more accurate predictions.
2.4. Step 4: Share Your Work And Build Your Online Presence
Once you’ve built a few data science projects, share them with others and build your online presence. This will help you showcase your skills, receive feedback, and connect with other data scientists.
2.4.1. Sharing Projects On GitHub
GitHub is a platform for hosting and sharing code. Upload your data science projects to GitHub to make them accessible to others.
- It makes you think about how to best present your projects, which is what you’d do in a data science role.
- They allow your peers to view your projects and provide feedback.
- They allow employers to view your projects.
2.4.2. Creating A Project Portfolio
A project portfolio is a collection of your data science projects that you can use to showcase your skills to potential employers.
- GitHub Repositories: Use your GitHub repositories as a portfolio.
- Personal Website: Create a personal website to showcase your projects and skills.
- Online Resume: Include your projects in your online resume or LinkedIn profile.
2.4.3. Starting A Simple Blog
Along with uploading your work to GitHub, you should also think about publishing a blog. When I was learning data science, writing blog posts helped me do the following:
- Capture interest from recruiters
- Learn concepts more thoroughly (the process of teaching really helps you learn)
- Connect with peers
2.4.4. Blog Post Topics
- Explaining data science and programming concepts
- Discussing your projects and walking through your findings
- Discussing how you’re learning data science
2.4.5. Visualizations And Examples
Create visualizations and examples to illustrate your blog posts. Visualizations can help readers understand complex concepts and make your blog posts more engaging.
2.5. Step 5: Learn From Others And Engage With The Community
After you’ve started to build an online presence, it’s a good idea to start engaging with other data scientists. You can do this in-person or in online communities.
2.5.1. Online Communities
- Dataquest Community: Dataquest has an online community that learners can use to receive feedback on projects, discuss tough data-related problems, and build relationships with data professionals.
- Kaggle Forums: Kaggle has a forum where you can ask questions, share insights, and collaborate with other data scientists.
- Reddit: Subreddits like r/datascience and r/machinelearning are popular online communities for data scientists.
- Stack Overflow: Stack Overflow is a question-and-answer website for programmers and data scientists.
2.5.2. Benefits Of Engaging In Online Communities
- Find other people to learn with
- Enhance your profile and find opportunities
- Strengthen your knowledge by learning from others
2.5.3. In-Person Meetups
Engage with people in-person through Meetups. In-person engagement can help you meet and learn from more experienced data scientists in your area.
2.6. Step 6: Continuously Push Your Boundaries
What kind of data scientists do companies want to hire? The ones that find critical insights that save them money or make their customers happier. You have to apply the same process to learning — keep searching for new questions to answer, and keep answering harder and more complex questions.
2.6.1. Striving For Continuous Improvement
If you look back on your projects from a month or two ago, and you don’t see room for improvement, you probably aren’t pushing your boundaries enough. You should be making strong progress every month, and your work should reflect that.
2.6.2. Ways To Push Your Boundaries
- Try working with a larger dataset
- Start a data science project that requires knowledge you don’t have
- Try making your project run faster
- Teach what you did in a project to someone else
2.6.3. Resources For Learning Data Science
- Online Courses: Platforms like Coursera, edX, and Udacity offer advanced data science courses.
- Books: “The Elements of Statistical Learning” by Hastie, Tibshirani, and Friedman and “Pattern Recognition and Machine Learning” by Bishop are classic textbooks on machine learning.
- Research Papers: Read research papers in data science and machine learning to stay up-to-date with the latest advancements.
3. Essential Tools And Technologies For Data Science
To excel in data science, it’s crucial to be proficient in a variety of tools and technologies. These tools enable you to efficiently collect, clean, analyze, and visualize data, as well as build and deploy machine learning models.
Category | Tool/Technology | Description |
---|---|---|
Programming Languages | Python | A versatile language with extensive libraries for data analysis, machine learning, and visualization. |
R | A language specifically designed for statistical computing and data analysis. | |
Data Manipulation | Pandas | A Python library for data manipulation and analysis, providing data structures like DataFrames for organizing and analyzing data. |
SQL | A language for querying and managing relational databases, allowing you to extract and manipulate data from databases. | |
Data Visualization | Matplotlib | A Python library for creating static, interactive, and animated visualizations in Python. |
Seaborn | A Python library for creating statistical graphics, providing a high-level interface for creating informative and visually appealing plots. | |
Tableau | A data visualization tool that allows you to create interactive dashboards and visualizations without writing code. | |
Machine Learning | Scikit-learn | A Python library for machine learning, providing tools for classification, regression, clustering, and model evaluation. |
TensorFlow | A deep learning framework developed by Google, used for building and training neural networks. | |
PyTorch | A deep learning framework developed by Facebook, known for its flexibility and ease of use. | |
Big Data | Hadoop | A framework for distributed storage and processing of large datasets. |
Spark | A fast and general-purpose cluster computing system for big data processing. | |
Cloud Computing | Amazon Web Services (AWS) | A suite of cloud computing services, including storage, computing, and machine learning. |
Microsoft Azure | A cloud computing platform offering a wide range of services, including data storage, machine learning, and artificial intelligence. | |
Google Cloud Platform (GCP) | A cloud computing platform providing a variety of services, including data analytics, machine learning, and data storage. | |
Version Control | Git | A distributed version control system for tracking changes in code and collaborating with others. |
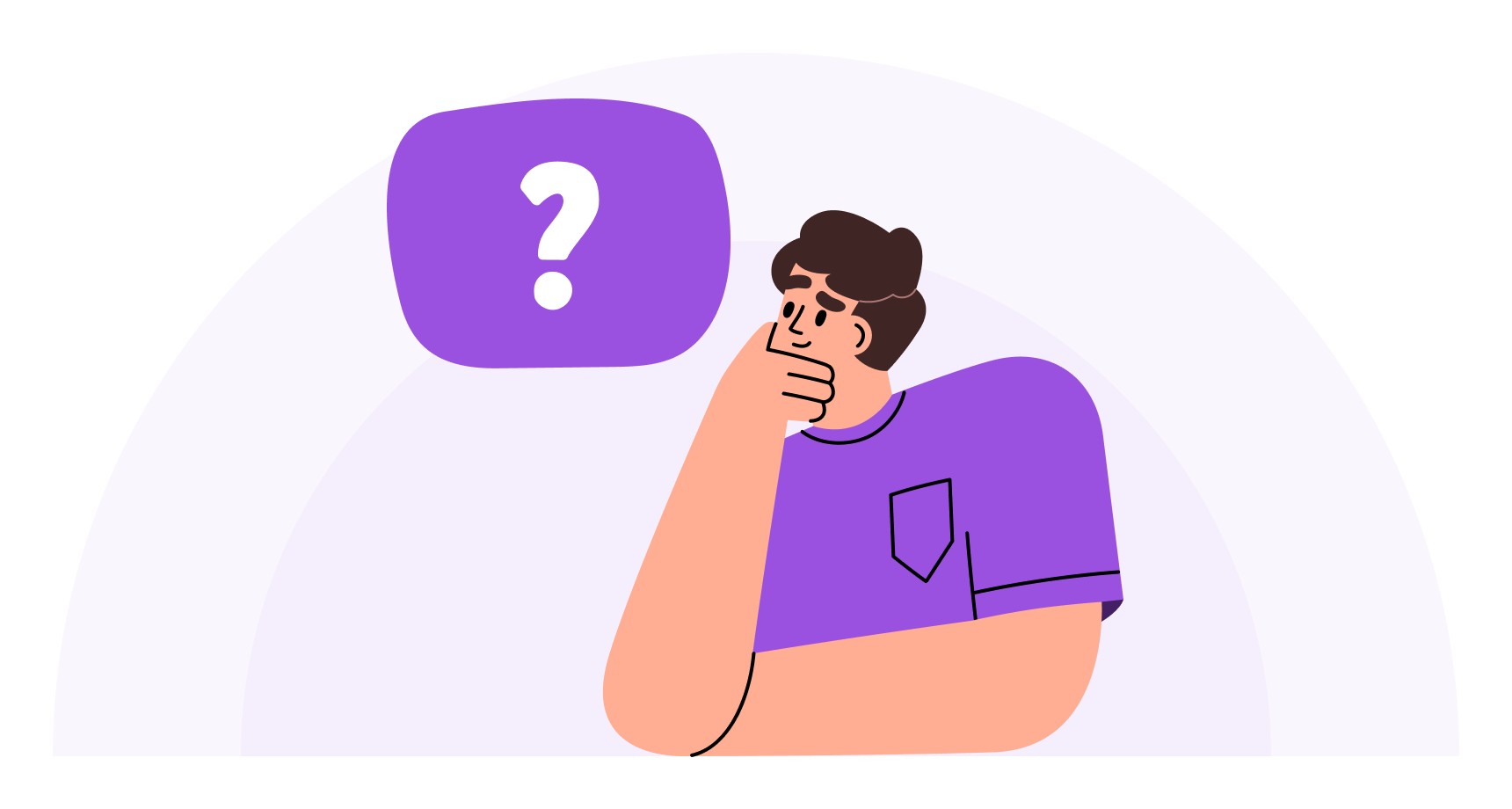
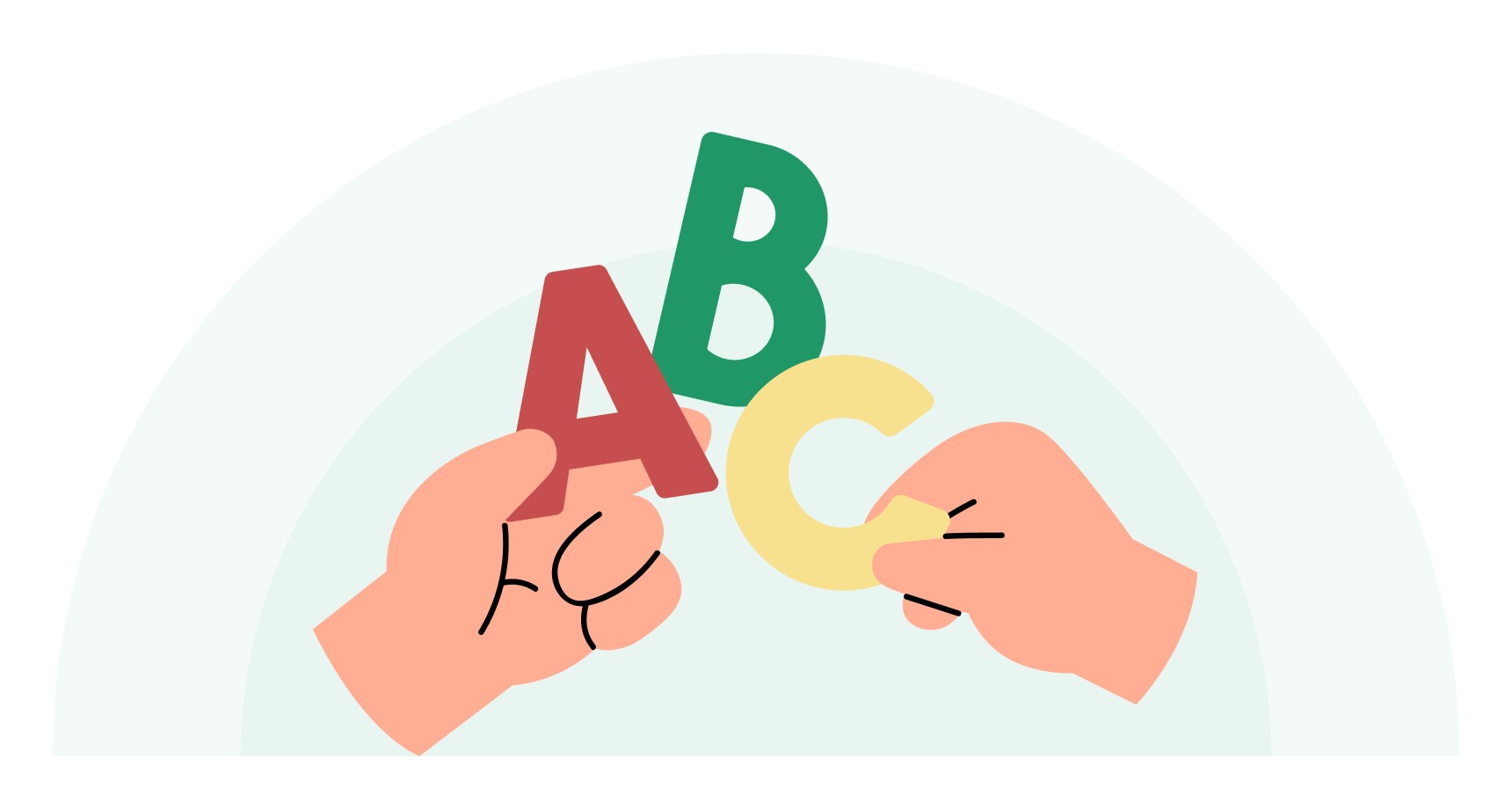
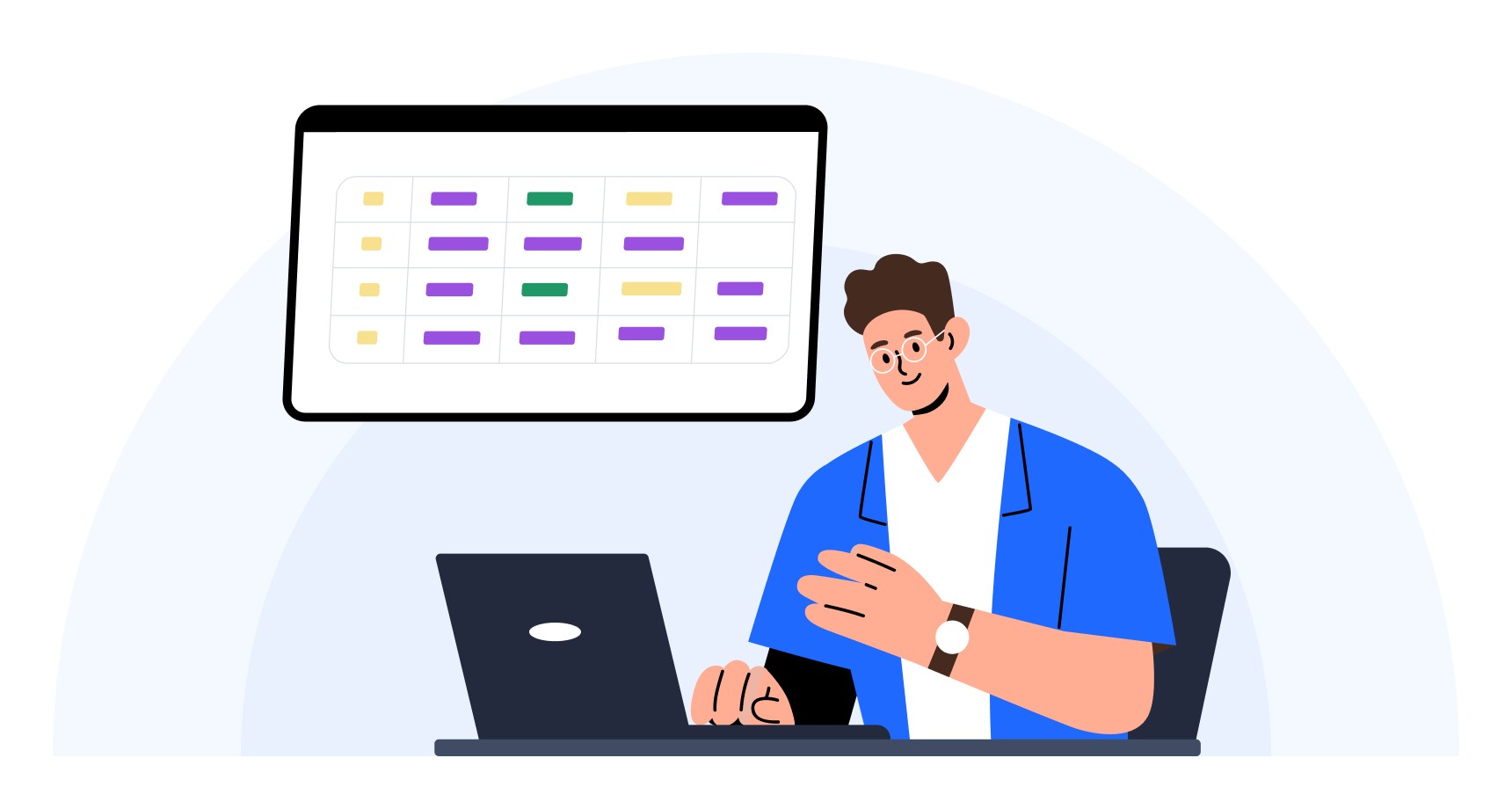
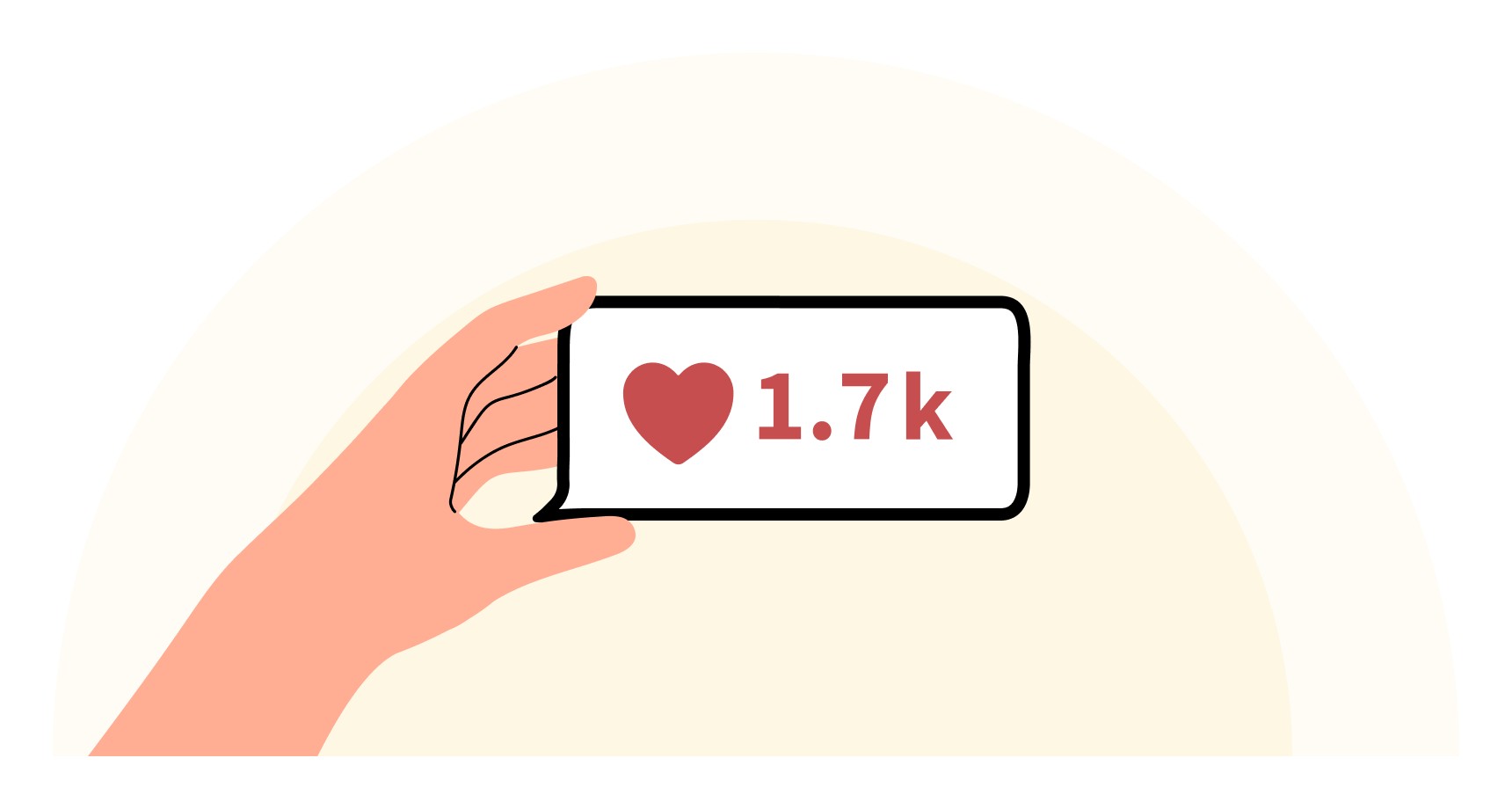
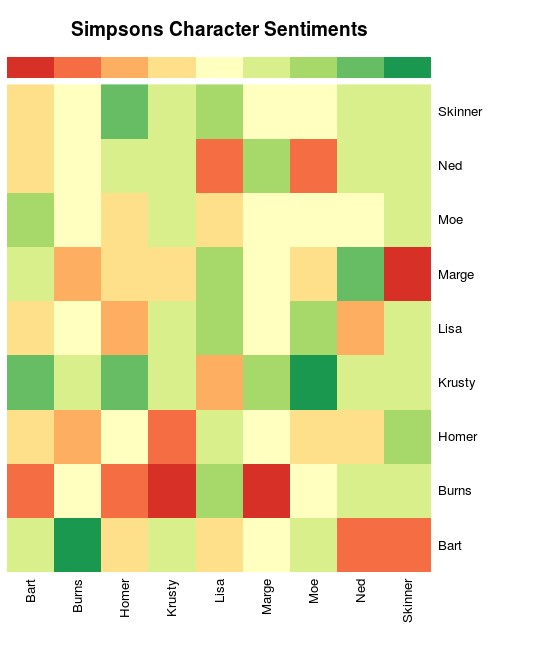
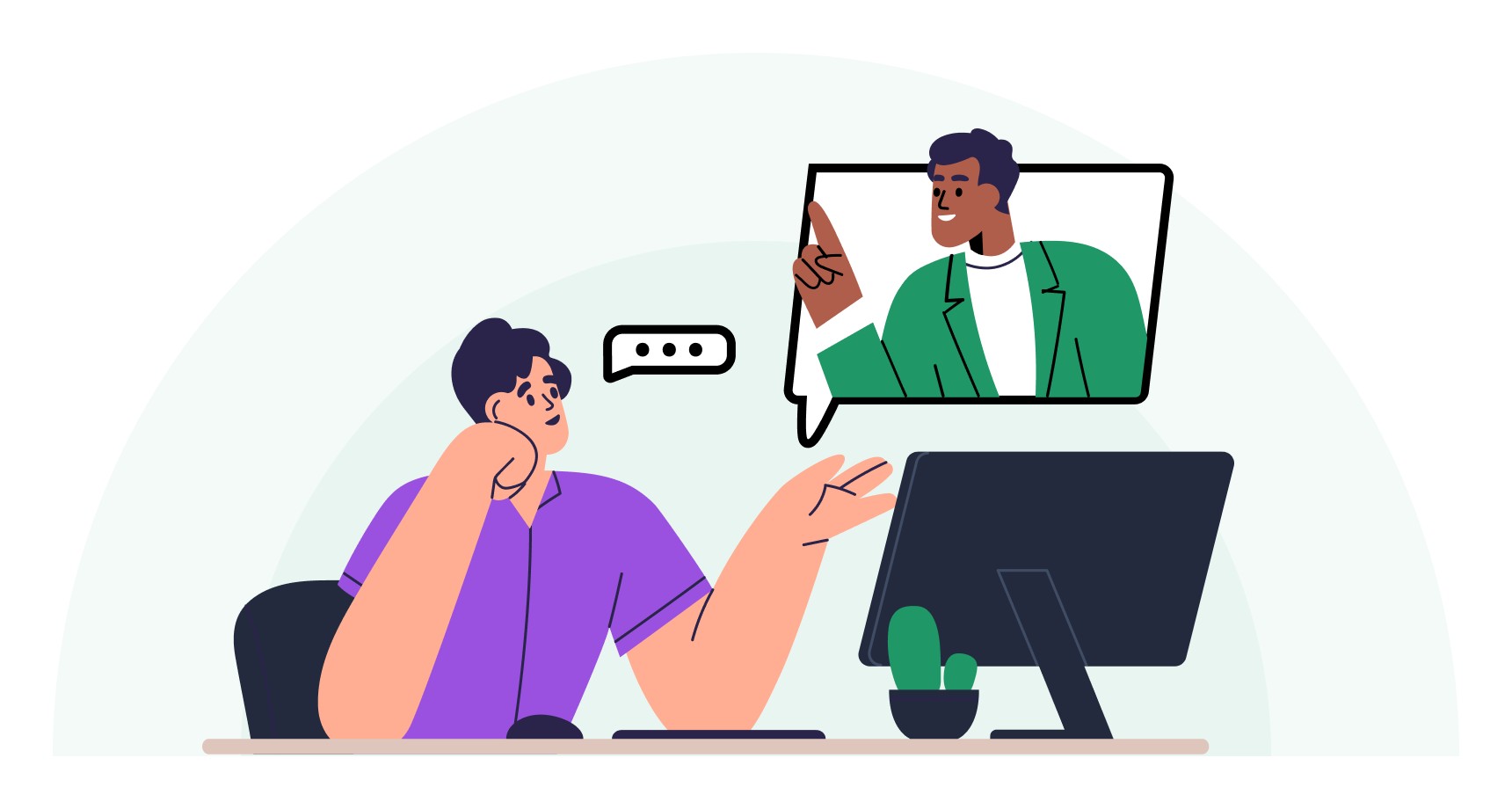
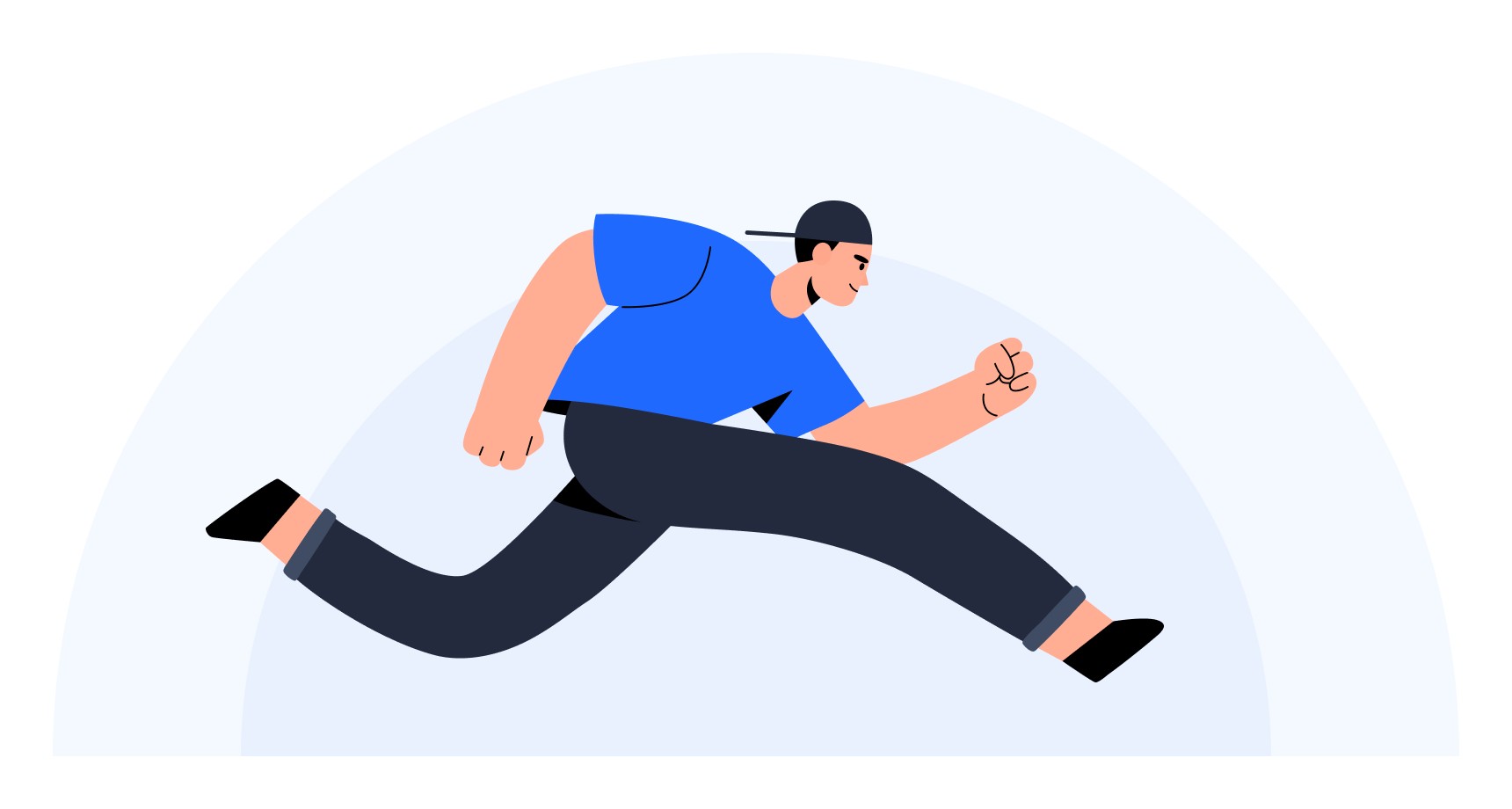
4. The Importance Of Continuous Learning And Adaptation
Data science is a rapidly evolving field, with new tools, technologies, and techniques emerging constantly. To stay competitive and relevant, it’s crucial to embrace continuous learning and adaptation.
4.1. Staying Up-To-Date With The Latest Advancements
- Read Research Papers: Follow the latest research in data science and machine learning to stay informed about new techniques and methodologies.
- Attend Conferences And Workshops: Participate in conferences and workshops to learn from experts, network with peers, and discover new trends in the field.
- Take Online Courses: Enroll in online courses to acquire new skills and deepen your understanding of specific topics.
4.2. Adapting To New Tools And Technologies
- Experiment With New Tools: Don’t be afraid to try out new tools and technologies to see how they can improve your workflow and enhance your capabilities.
- Seek Out Training: Take advantage of training opportunities to learn how to use new tools and technologies effectively.
- Contribute To Open Source Projects: Contributing to open source projects is a great way to learn new tools and technologies and collaborate with other developers.
4.3. The Role Of LEARNS.EDU.VN In Your Data Science Journey
LEARNS.EDU.VN is committed to providing you with the resources and support you need to succeed in data science. Our comprehensive courses, expert guidance, and community forums will help you acquire the skills, knowledge, and connections you need to thrive in this exciting field.
- Structured Learning Paths: Our structured learning paths will guide you through the essential concepts and skills you need to become a data scientist.
- Hands-On Projects: Our hands-on projects will give you the opportunity to apply your skills and build a portfolio of work.
- Expert Guidance: Our expert instructors will provide you with personalized guidance and support.
- Community Forums: Our community forums will connect you with other learners and data science professionals.
5. Addressing Common Challenges And Concerns
Embarking on a data science career can be both exciting and challenging. It’s common to encounter obstacles and have concerns along the way. Let’s address some of the most frequently asked questions and concerns about learning data science.
5.1. Overcoming The Fear Of Math And Statistics
Many aspiring data scientists are intimidated by the math and statistics involved in the field. While a strong foundation in these areas is certainly beneficial, it’s not always a prerequisite for success.
- Focus On Practical Applications: Start by focusing on the practical applications of math and statistics in data science. Learn how these concepts are used to solve real-world problems and gain insights from data.
- Utilize Online Resources: There are numerous online resources available to help you learn math and statistics, including Khan Academy, Coursera, and edX.
- Seek Help When Needed: Don’t hesitate to ask for help from instructors, mentors, or online communities when you’re struggling with a particular concept.
5.2. Balancing Learning With Other Commitments
Many aspiring data scientists have other commitments, such as work, family, or school. Balancing learning with these commitments can be challenging, but it’s certainly possible.
- Set Realistic Goals: Set realistic learning goals that fit your schedule and commitments. Don’t try to learn everything at once.
- Create A Study Schedule: Create a study schedule and stick to it as much as possible.
- Utilize Time Management Techniques: Use time management techniques, such as the Pomodoro Technique, to stay focused and productive.
- Take Advantage Of Online Learning Platforms: Online learning platforms offer flexible learning options that allow you to learn at your own pace.
5.3. Staying Motivated And Avoiding Burnout
Learning data science can be a long and challenging process, and it’s easy to lose motivation or experience burnout.
- Set Clear Goals: Set clear learning goals and track your progress to stay motivated.
- Celebrate Your Accomplishments: Celebrate your accomplishments, no matter how small they may seem.
- Take Breaks: Take regular breaks to avoid burnout.
- Find A Community: Connect with other data scientists to share your experiences and receive support.
- Work On Projects You’re Passionate About: Working on projects you’re passionate about will help you stay motivated and engaged.
5.4. Understanding The Evolving Role Of Data Science
As AI and machine learning continue to advance, the role of data scientists is evolving. Data scientists are increasingly collaborating with AI engineers and machine learning specialists to develop and deploy AI-powered solutions.
- Acquire New Skills: Stay up-to-date with the latest advancements in AI and machine learning and acquire the skills needed to work with these technologies.
- Collaborate With Others: Collaborate with AI engineers, machine learning specialists, and other data professionals to leverage their expertise and insights.
- Focus On Strategic Analysis: Focus on strategic analysis and problem-solving, leaving the more repetitive tasks to AI-powered tools.
6. Building A Successful Data Science Career
Becoming a data scientist is just the first step. To build a successful data science career, you need to develop a range of skills, gain experience, and network with other professionals.
6.1. Developing Essential Skills
- Technical Skills: Master the essential tools and technologies for data science, including Python, R, SQL, and machine learning libraries.
- Analytical Skills: Develop strong analytical skills to effectively analyze data, identify patterns, and draw meaningful conclusions.
- Communication Skills: Hone your communication skills to effectively communicate your findings to technical and non-technical audiences.
- Problem-Solving Skills: Develop strong problem-solving skills to tackle complex data science challenges.
- Business Acumen: Gain a solid understanding of business principles to align your data science work with organizational goals.
6.2. Gaining Practical Experience
- Internships: Pursue internships to gain practical experience in data science.
- Personal Projects: Work on personal projects to showcase your skills and build a portfolio of work.
- Freelance Work: Take on freelance data science projects to gain experience and build your reputation.
- Open Source Contributions: Contribute to open source data science projects to collaborate with other professionals and enhance your skills.
6.3. Networking And Building Connections
- Attend Industry Events: Attend industry conferences, workshops, and meetups to network with other data science professionals.
- Join Online Communities: Join online communities like Kaggle, Reddit, and Stack Overflow to connect with other data scientists.
- Connect On LinkedIn: Connect with data science professionals on LinkedIn to build your network.
- Attend Networking Events: Attend networking events to meet potential employers and learn about job opportunities.
6.4. Crafting A Compelling Resume And Portfolio
- Highlight Relevant Skills: Highlight your relevant skills and experiences on your resume.
- Showcase Projects: Showcase your data science projects in your portfolio.
- Quantify Achievements: Quantify your achievements whenever possible.
- Tailor Your Resume: Tailor your resume to each job you apply for.
6.5. Preparing For Data Science Interviews
- Practice Technical Questions: Practice answering technical questions about data science concepts and tools.
- Prepare Behavioral Questions: Prepare answers to behavioral questions about your skills, experiences, and motivations.
- Research The Company: Research the company and the role you’re applying for.
- Ask Questions: Ask thoughtful questions at the end of the interview to show your interest.
7. The Future Of Data Science: Emerging Trends And Opportunities
Data science is a dynamic field that is constantly evolving. As new technologies and techniques emerge, new opportunities arise for data scientists.
7.1. The Rise Of Explainable AI (XAI)
Explainable AI (XAI) is a growing trend in data science that focuses on making AI models more transparent and interpretable. XAI techniques allow data scientists to understand how AI models make decisions, which is crucial for building trust and ensuring ethical AI practices.
7.2. The Growth Of Edge Computing
Edge computing involves processing data closer to the source, rather than sending it to a central data center. This can improve performance, reduce latency, and enhance security. Data scientists are increasingly working with edge computing technologies to develop and deploy AI-powered applications in areas like IoT, autonomous vehicles, and healthcare.
7.3. The Increasing Importance Of Data Privacy And Security
As data becomes more valuable, data privacy and security are becoming increasingly important. Data scientists need to be aware of data privacy regulations and security best practices to ensure that data is handled responsibly and ethically.
7.4. The Convergence Of Data Science And Other Disciplines
Data science is increasingly converging with other disciplines, such as healthcare, finance, and engineering. This convergence is creating new opportunities for data scientists to apply their skills to solve complex problems in these fields.
7.5. How LEARNS.EDU.VN Prepares You For The Future
LEARNS.EDU.VN is committed to providing you with the skills and knowledge you need to thrive in the future of data science. Our curriculum is constantly updated to reflect the latest trends and technologies.
- AI And Machine Learning Focus: Our courses emphasize AI and machine learning, preparing you for the evolving role of data scientists.
- Ethical Data Practices: We teach ethical data practices to ensure that you handle data responsibly and ethically.
- Industry-Relevant Skills: We equip you with the industry-relevant skills you need to succeed in data science careers.
- Continuous Learning Resources: We provide continuous learning resources to help you stay up-to-date with the latest advancements in the field.
8. Conclusion: Embracing The Journey To Data Science Mastery
Embarking on a data science journey is a challenging but rewarding endeavor. With the right mindset, skills, and resources, anyone can learn data science and build a successful career in this exciting field. Remember to focus on continuous learning, adapt to new technologies, and embrace the challenges that come your way. And with the guidance and support of LEARNS.EDU.VN, you’ll have the resources and connections you need to achieve your data science goals.
Studying to become a data scientist or data engineer isn’t easy, but the key is to stay motivated and enjoy what you’re doing. If you’re consistently building projects and sharing them, you’ll build your expertise and get the data scientist job that you want. Anyone can become a data scientist if you’re motivated enough.
After years of being frustrated with how conventional sites taught data science, I created Dataquest, a better way to learn data science online. Dataquest solves the problems of MOOCs, where you never know what course to take next, and you’re never motivated by what you’re learning.
Dataquest leverages the lessons I’ve learned from helping thousands of people learn data science, and it focuses on making the learning experience engaging. At Dataquest, you’ll build dozens of projects, and you’ll learn all the skills you need to be a successful data scientist. Dataquest students have been hired at companies like Accenture and SpaceX.
We wish you all the best on your path to becoming a data scientist!
Ready to unlock your potential and embark on a data science journey? Visit LEARNS.EDU.VN today to explore our comprehensive courses and expert guidance!
Contact Us:
- Address: 123 Education Way, Learnville, CA 90210, United States
- Whatsapp: +1 555-555-1212
- Website: LEARNS.EDU.VN
9. Frequently Asked Questions (FAQs)
9.1. What is data science and why is it important?
Data science is an interdisciplinary field that uses scientific methods, processes, algorithms, and systems to extract knowledge and insights from structured and unstructured data. It’s important because it enables organizations to make data-driven decisions, improve efficiency, and gain a competitive advantage.
9.2. What skills do I need to become a data scientist?
Essential skills for data scientists include programming (Python, R), data manipulation (SQL, Pandas), data visualization (Matplotlib, Seaborn), machine learning (Scikit-learn, TensorFlow), and strong analytical and communication skills.
9.3. How long does it take to learn data science?
The time it takes to learn data science varies depending on your background, learning style, and dedication. It can take anywhere from several months to a few years to become proficient.
9.4. What are the best resources for learning data science?
Excellent resources for learning data science include online courses (Coursera, edX, Dataquest), books, research papers, and online communities (Kaggle, Reddit).
9.5. Do I need a degree to become a data scientist?
While a degree in a related field (computer science, statistics, mathematics) can be beneficial, it’s not always required. Many data scientists come from diverse backgrounds and have acquired their skills through self-study and online resources.
9.6. What is the job outlook for data scientists?
The job outlook for data scientists is excellent. The U.S. Bureau of Labor Statistics projects employment in computer and information research science occupations, including data science, to grow 23 percent from 2022 to 2032.
9.7. What is the average salary for a data scientist?
The average salary for a data scientist varies depending on experience, location, and industry. According to Glassdoor, the national average salary for a Data Scientist is $129,444 in the United States.
9.8. What are the different roles in data science?
Different roles in data science include data scientist, data analyst, machine learning engineer, data engineer, and business intelligence analyst.
9.9. What are some common data science projects?
Common data science projects include predicting customer churn, analyzing customer sentiment, detecting fraud, and forecasting sales.
9.10. How can LEARNS.EDU.VN help me learn data science?
learns.edu.vn offers comprehensive courses, expert guidance, and community forums to help you acquire the skills, knowledge, and connections you need to succeed in data science.