Natural Language Processing (NLP) and Machine Learning (ML) are often mentioned together, leading to confusion about their distinct roles. This guide from LEARNS.EDU.VN clarifies How Is Nlp Different From Machine Learning, providing a deep dive into their unique characteristics, applications, and synergistic relationship. Understand the nuances of these AI subfields and unlock the power to leverage both for innovative solutions. Explore our comprehensive resources for advanced insights into AI-driven technologies, data analysis, and predictive modeling.
1. Understanding Machine Learning
Machine learning (ML) represents a transformative approach to data analysis, automating the creation of analytical models. At its core, ML operates on the principle that systems can evolve through data, discern patterns, and autonomously arrive at decisions without direct human intervention. This branch of Artificial Intelligence (AI) has seen unparalleled growth, becoming a highly sought-after expertise in today’s tech-driven landscape.
A quintessential example is Netflix’s recommendation system, a testament to the power and precision of ML algorithms. By scrutinizing vast datasets of viewing habits, Netflix crafts personalized suggestions, aligning user preferences with similar viewers’ choices.
In today’s competitive landscape, businesses must leverage AI for effective personalization. By analyzing every available customer interaction and touchpoint, businesses can develop a more nuanced understanding of customer motivations and behavior. According to a study by McKinsey, companies that excel at personalization generate 40% more revenue than those that don’t.
Key Takeaways:
- ML automates analytical model creation.
- Systems learn from data without human intervention.
- Netflix’s recommendation system is a prime example.
- Personalization driven by AI is crucial for business success.
2. Unveiling Natural Language Processing
Natural language processing (NLP) empowers machines to comprehend and interpret human language, transcending mere text reading. NLP-driven systems analyze written or spoken language, enabling tasks like speech recognition, sentiment analysis, and automated text summarization.
Imagine interacting with Alexa. When posed with a query, Alexa utilizes NLP to decode your words and then employs it again to formulate a response that is both coherent and human-friendly.
Consider an e-commerce clothing brand that allows customers to meet up online and shop together. NLP could generate lifelike audio responses, complete with linguistic nuances and tone modulation. NLP could even automatically translate these responses into multiple languages. These capabilities can be extremely useful for global businesses.
It is vital to note that virtual reality and NLP may not suit every e-commerce business, so it’s worth familiarizing yourself with the best practices.
Key Takeaways:
- NLP enables machines to understand human language.
- Applications include speech recognition and sentiment analysis.
- Alexa exemplifies NLP in action.
- NLP can enhance virtual shopping experiences.
3. 7 Key Differences Between Machine Learning and NLP
While both Machine Learning and Natural Language Processing are subsets of Artificial Intelligence, they operate with distinct methodologies and objectives. Understanding their differences is crucial for selecting the appropriate technique for specific tasks. Here’s a comprehensive breakdown:
Feature | Machine Learning | Natural Language Processing |
---|---|---|
Definition | A subset of AI that enables machines to learn from data without explicit programming. | A subset of AI focused on enabling computers to understand, interpret, and generate human language. |
Core Objective | To identify patterns in data and make predictions based on those patterns. | To enable computers to comprehend and interact with human language in a meaningful way. |
Primary Applications | Recommendation systems, search algorithms, fraud detection, predictive analytics. | Chatbots, sentiment analysis, language translation, grammar checking, speech recognition. |
Methodologies | Supervised learning (training on labeled data), unsupervised learning (finding patterns in unlabeled data), reinforcement learning (learning through trial and error). | Syntactic analysis (analyzing sentence structure), semantic analysis (understanding meaning), natural language generation (creating human-like text). |
Focus | Accuracy and pattern recognition in data. | Understanding and processing human language. |
Data Requirements | Requires large amounts of data to capture complex relationships. | Requires machine learning techniques for accurate and automated processing. |
Adaptability | Requires continuous monitoring and maintenance to ensure performance. | Limited adaptability to new domains and problems due to task-specific design. |
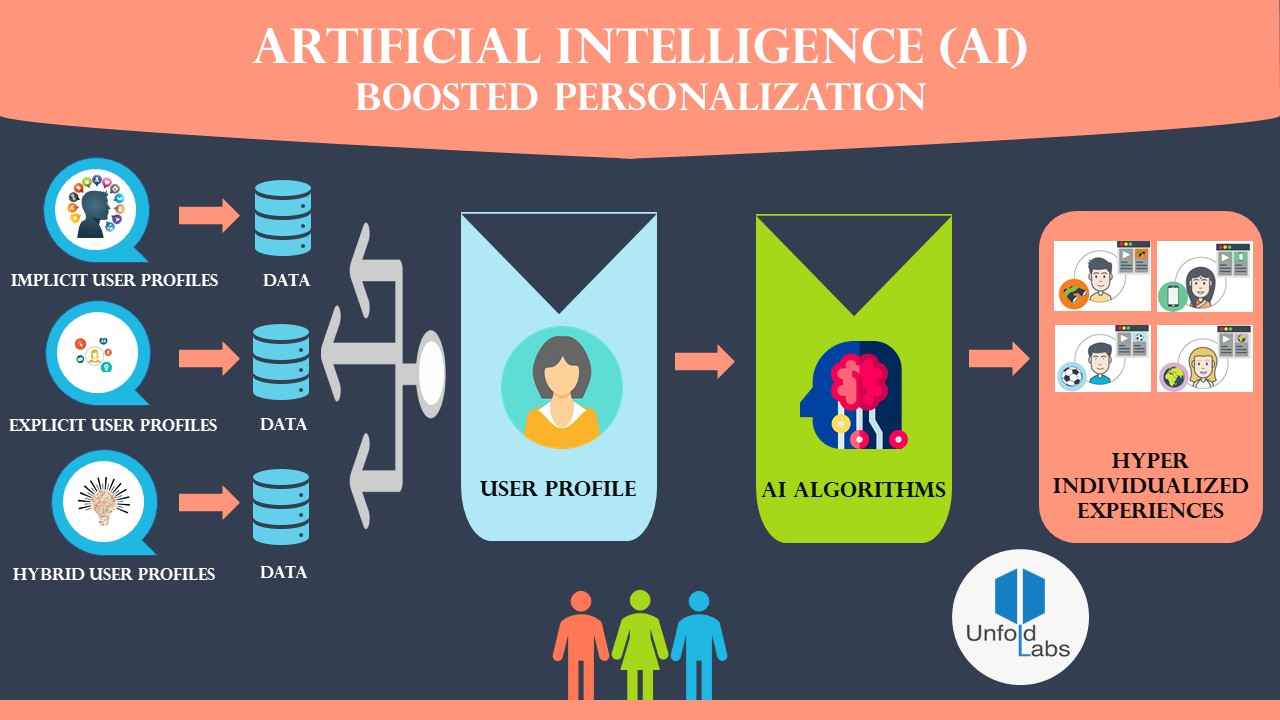
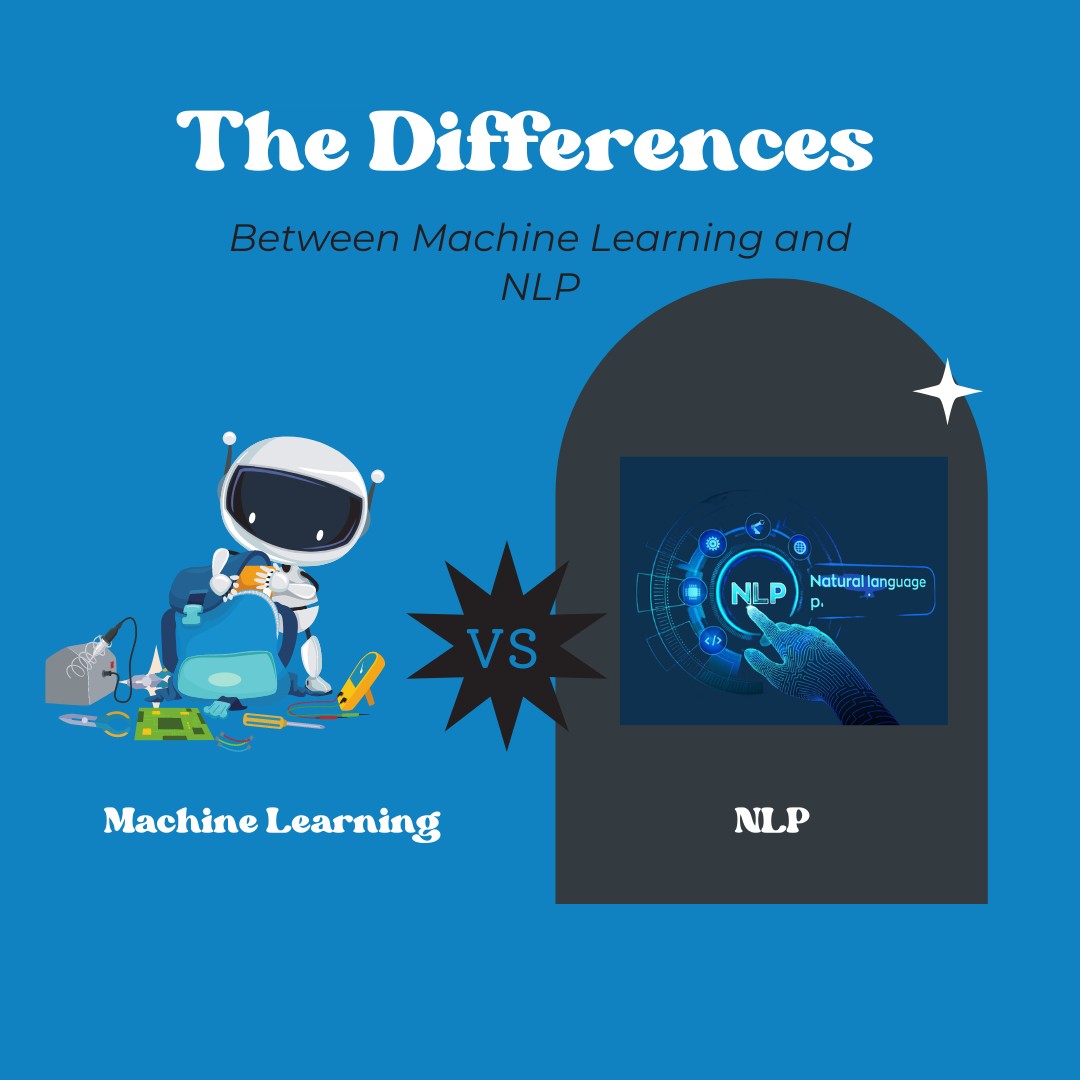
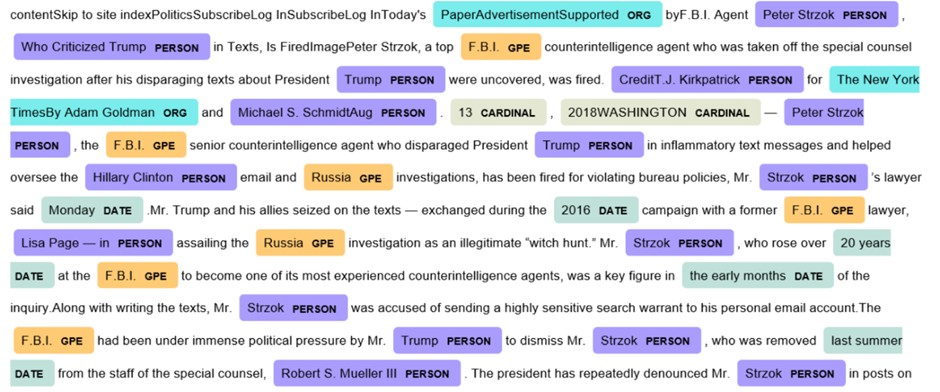
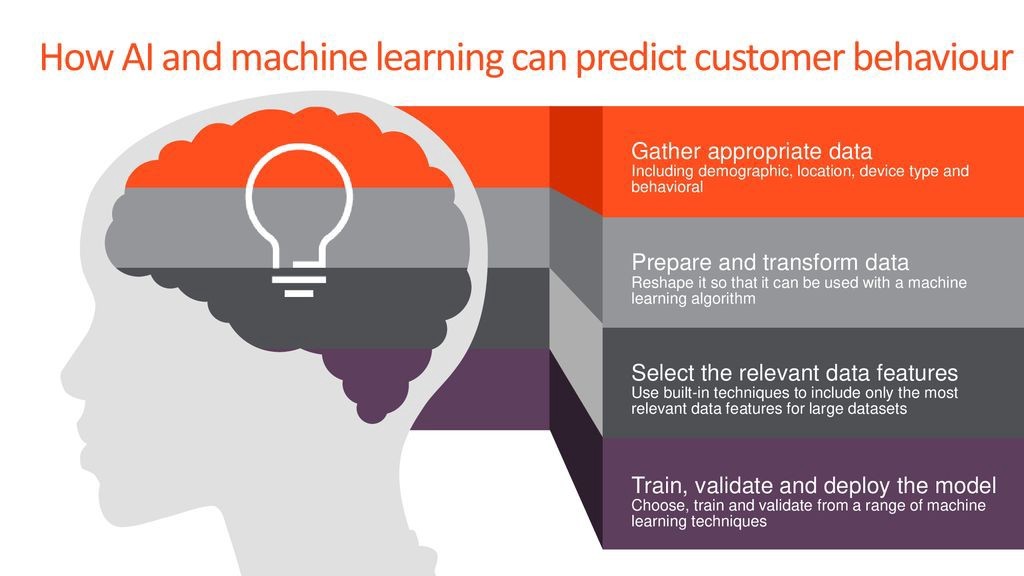
Key Insights:
- Machine learning excels at identifying patterns in data and making predictions, requiring large datasets for effective training.
- NLP is designed to enable computers to understand and interact with human language, employing syntactic and semantic analysis.
- While machine learning focuses on accuracy and pattern recognition, NLP is concerned with understanding and processing human language.
- Machine learning applications include recommendation systems and fraud detection, whereas NLP is used in chatbots and language translation.
4. Real-World Applications of NLP
NLP has revolutionized numerous industries by enabling machines to understand and process human language. Here are some prominent use cases:
4.1 Grammar Checker
Grammar checking is a popular application of NLP. Grammar checkers detect and correct grammatical mistakes in the input text. This is especially useful for bloggers, social media managers, and anyone who wants to ensure their writing is clear and professional.
While manual reviews are still possible, grammar checkers offer speed and accuracy. It provides suggestions for correcting these errors. According to Grammarly, 76% of users find that using their tool significantly improves their writing skills.
If you’re looking to automate some of your blog content or social media posts, it’s worth getting yourself familiarized with AI for social media, as it can help you achieve incredible results.
4.2 Sentiment Analysis
Sentiment analysis involves identifying positive or negative feelings in a sentence. It helps analyze customer reviews, judge mood via written text or voice analysis, and perform other similar tasks. Companies can gain insight into how customers feel about brands or products by analyzing social media posts, product reviews, or online surveys.
For example, you could analyze survey results from a recently concluded webinar to detect positive or sarcastic comments from audiences. Research indicates that companies utilizing sentiment analysis can improve customer satisfaction by up to 20%.
4.3 Named Entity Recognition
This process identifies information units such as names, organizations, locations, and numeric expressions from unstructured text. Understanding and analyzing customer demands and behavior is essential for e-commerce businesses.
Using natural language processing, machines can understand what phrases and words users use when searching for products. This allows users who use a search engine to customize their searches when using the system. Customers shop online because they want their shopping experience to get better. The priority for improvement would be product discovery (including searches and category browsing), since it can always help customers find products. However, customers are generally hard to impress. Even when the search queries are not specific, most customers expect the search systems to recognize their intent.
Key Highlights:
- Grammar Checkers enhance writing quality and professionalism.
- Sentiment Analysis provides insights into customer opinions and emotions.
- Named Entity Recognition is essential for understanding customer demands and improving search accuracy.
5. Practical Applications of Machine Learning
Machine Learning is transforming various sectors, offering powerful tools for automation, prediction, and decision-making. Here are key applications:
5.1 Real-Time Chatbots
Chatbots were one of the first forms of automation that allowed people to communicate with machines that can perform actions based on human requests or requirements. AI-powered chatbots are now designed to handle more complex requests, making conversational experiences more intuitive.
For example, imagine a startup trying to sell affiliate tracking software to small businesses. Chatbots can assist in gathering customer data and determining the next steps. These chatbots can also schedule meetings with the appropriate experts and recommend solutions like real humans. Studies show that businesses using AI-powered chatbots can reduce customer service costs by up to 30%.
5.2 Fraud Detection
The ability of machine learning to understand patterns and detect anomalies that fall outside of those patterns makes it a valuable tool for detecting fraudulent activity. It can be used to learn about a specific customer’s typical behavior, such as when and where they use their credit card.
Machine learning can use that information and other data sets to determine which transactions are within the normal range accurately and legitimately. They can also determine which transactions are outside expected norms—and therefore likely fraudulent—in milliseconds.
5.3 Predicting Behavior
Machine learning models can be used by businesses to predict customer behavior based on historical data. Companies search social media for what people are talking about and then identify those looking for a specific product or service.
Let’s imagine a marketer who wants to write a blog post but isn’t sure whether to write about creating viral SaaS product growth or the average affiliate commission rate. AI can assist the marketer in generating hundreds of keywords with a few clicks. This allows the marketer to screen for ideas that the audience is most interested in, prioritize content, and visualize internal linking opportunities for SEO benefits.
Key Advantages:
- Real-Time Chatbots improve customer interaction and reduce costs.
- Fraud Detection enhances security and protects against financial losses.
- Predicting Behavior enables targeted marketing and content creation.
6. Making Sense of Machine Learning and NLP
Initially, there is some overlap between machine learning and NLP, as machine learning is frequently used as a tool for NLP tasks. We can say that NLP is a subset of machine learning that enables computers to understand, analyze, and generate human language. If you have a large amount of written data and want to gain some insights, you should learn and use NLP.
However, machine learning is not required to learn NLP because there are other things you’ll need, such as NER (named entity recognizer), POS Tagged (a parts of speech tagger can identify nouns, verbs, and other parts of speech tags in text). However, to use NLP effectively, you’ll need machine learning.
So, machine learning is definitely worth learning, and more online businesses are incorporating it into their operations, with the more established ones leading the way.
For example, machine learning assists software developers in predicting whether a software release will contain a large or small number of bugs. This can significantly assist release managers in better planning their software release cycle based on the resources available. Above all, keep in mind that these new technologies are a part of our lives and here to stay.
Key Considerations:
- NLP often relies on machine learning for enhanced capabilities.
- Machine learning is beneficial for predicting software release bugs.
- Both NLP and machine learning are crucial for staying competitive.
7. LEARNS.EDU.VN: Your Gateway to Mastering AI Technologies
At LEARNS.EDU.VN, we understand the growing demand for expertise in AI technologies like Machine Learning and Natural Language Processing. We provide a comprehensive platform for individuals and professionals looking to enhance their skills and knowledge in these cutting-edge fields.
7.1 Comprehensive Courses and Resources
LEARNS.EDU.VN offers a wide range of courses tailored to various skill levels. From introductory courses that demystify the basics of AI to advanced programs that delve into complex algorithms and applications, we have something for everyone. Our resources include:
- In-depth articles and tutorials: Covering fundamental concepts, practical applications, and the latest trends in AI.
- Hands-on projects: Allowing you to apply your knowledge and build a portfolio of AI projects.
- Expert instructors: Providing guidance and mentorship throughout your learning journey.
7.2 Tailored Learning Paths
Whether you are a student, a working professional, or an enthusiast, LEARNS.EDU.VN provides tailored learning paths to help you achieve your specific goals. Our curriculum is designed to address the challenges faced by our audience, such as:
- Difficulty finding reliable learning resources: We curate high-quality content from trusted sources and industry experts.
- Lack of motivation and direction: Our structured learning paths and supportive community keep you engaged and motivated.
- Complex concepts: We simplify complex ideas with clear explanations and real-world examples.
7.3 Cutting-Edge Content
Stay up-to-date with the latest advancements in AI through LEARNS.EDU.VN. We continuously update our content to reflect the newest technologies, techniques, and best practices. Our platform provides insights into:
- Advanced educational methods: Discover the most effective ways to learn AI concepts.
- Online learning trends: Explore how online education is evolving and adapting to meet the demands of the AI field.
- Innovative tools and applications: Learn about the latest AI tools and how to use them effectively.
By providing comprehensive and up-to-date information, LEARNS.EDU.VN ensures that you have the knowledge and skills to excel in the field of AI.
8. Latest Innovations in AI and Education
The landscape of AI and education is constantly evolving, with new tools and techniques emerging regularly. Here’s a look at some of the latest innovations:
Innovation | Description | Educational Application |
---|---|---|
AI-Powered Tutoring Systems | Intelligent systems that provide personalized instruction and feedback to students based on their learning needs. | Personalized learning experiences, adaptive assessments, and targeted interventions to improve student outcomes. |
Automated Content Creation | AI tools that can generate educational content, such as quizzes, lesson plans, and study guides, reducing the workload for educators. | Efficient content creation, customized learning materials, and enhanced student engagement. |
AI-Driven Assessment Tools | Systems that use AI to evaluate student performance, provide feedback, and identify areas for improvement. | Accurate and objective assessment, timely feedback, and data-driven insights for educators to tailor their instruction. |
Virtual and Augmented Reality | Immersive technologies that create interactive and engaging learning environments. | Enhanced visualization of complex concepts, virtual field trips, and interactive simulations to improve student understanding and retention. |
Natural Language Processing in Education | NLP tools that can analyze student writing, provide feedback on grammar and style, and detect plagiarism. | Improved writing skills, enhanced critical thinking, and academic integrity. |
These innovations are transforming the way we learn and teach, making education more accessible, personalized, and effective.
9. Frequently Asked Questions (FAQ) About NLP and Machine Learning
1. What is the primary difference between NLP and Machine Learning?
NLP focuses on enabling computers to understand and process human language, while Machine Learning is about enabling machines to learn from data to make predictions or decisions.
2. Can NLP exist without Machine Learning?
Yes, but to a limited extent. NLP can use rule-based systems, but Machine Learning significantly enhances NLP’s accuracy and capabilities.
3. Is Machine Learning a subset of NLP, or vice versa?
NLP is generally considered a subset of AI, with Machine Learning often used as a tool within NLP.
4. What are the main applications of NLP?
Chatbots, language translation, sentiment analysis, grammar checking, and speech recognition.
5. What are the main applications of Machine Learning?
Recommendation systems, fraud detection, predictive analytics, and image recognition.
6. Do I need a lot of data to use NLP or Machine Learning effectively?
Machine Learning typically requires large amounts of data, while NLP’s data requirements depend on the specific task.
7. How does sentiment analysis work in NLP?
Sentiment analysis uses NLP techniques to identify and classify the emotional tone (positive, negative, neutral) in text.
8. What skills are required to learn NLP and Machine Learning?
Basic programming knowledge, understanding of statistics, and familiarity with algorithms are beneficial.
9. How can businesses benefit from NLP?
By improving customer service, automating tasks, gaining insights from customer feedback, and enhancing marketing efforts.
10. How can businesses benefit from Machine Learning?
By predicting customer behavior, detecting fraud, optimizing processes, and making data-driven decisions.
10. Call to Action
Ready to dive deeper into the world of AI? Explore the comprehensive courses and resources available at LEARNS.EDU.VN. Whether you’re looking to master machine learning, natural language processing, or both, our expert-led programs will equip you with the skills and knowledge to excel. Don’t miss out on the opportunity to transform your career and innovate in the field of artificial intelligence. Visit LEARNS.EDU.VN today and start your AI journey!
Contact Us:
- Address: 123 Education Way, Learnville, CA 90210, United States
- WhatsApp: +1 555-555-1212
- Website: learns.edu.vn